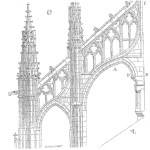
We’ve collected data and it’s time for the analysis.
As you may recall, in the last article on Planning a Taguchi L4 Array Experiment, we drafted a set of four prototypes. The specific arrangement of factors and levels will now allow us to analyze each factor separately.
The intent is to find the optimal level or setting for each factor, plus which is the most important factor.
The data
The website equally directs each visitor to one of the four prototype pages.
If the visitor then joined the site, we counted that as a conversion. We gathered data on 2,000 visitors, 500 to each page, and counted conversions.
The following table has the count of conversions per run (prototype page).
Run |
A | B | C | Y |
1 |
1 | 1 | 1 | 48 |
2 |
1 | 2 | 2 |
32 |
3 | 2 | 1 | 2 |
22 |
4 | 2 | 2 | 1 |
33 |
I’ve included the L4 array as we will use the level assignments shortly for the analysis.
Note Run 1 did much better than the other runs and while tempted to just implement the page configuration of Run 1, we may be missing an even better configuration.
Remember that the experiment only has four of the eight possible combinations of factors and levels.
The L4 array math
The first step is to isolate each factor with an average response, Y’s, for each level.
Factor |
level | Sum Y | Y-bar | MSD |
S/N |
A |
A1 | 48 + 32 | 40 | ||
A2 | 22 + 33 | 27.5 | |||
total |
67.5 |
||||
B |
B1 | 48 + 22 | 35 | ||
B2 | 32 + 33 |
32.5 |
|||
total | 67.5 | ||||
C | C1 | 48 + 33 |
40.5 |
||
C2 |
32 + 22 | 27 | |||
total |
67.5 |
The mean response, for each factor, is the tally of run responses, Y’s, for the runs containing that factor.
The counts to sum for each factor correspond to the L4 array. The two runs that contained level 1 for factor A are Run 1 and Run 2, corresponding to the 1’s under column A.
All four run responses are tallied slightly differently for each factor.
For example, for factor A, level A1 has the responses from Run 1 (48) and Run 2 (32). Level A2 has counts from Run 3 and Run 4.
The ‘total’ rows are a check that you have all four responses for each factor.
The next step is to calculate the mean square deviation (MSD).
Depending on the objective of the experiment select the appropriate formula.
In this case, we seek the maximum setting to achieve high conversion rates, thus will use the MSD formula for bigger is better, B-type, is.
$$ \large\displaystyle MSD=\frac{{}^{1}\!\!\diagup\!\!{}_{Y_{1}^{2}}\;+{}^{1}\!\!\diagup\!\!{}_{Y_{2}^{2}}\;+\cdots +{}^{1}\!\!\diagup\!\!{}_{Y_{n}^{2}}\;}{n}$$
The formula for smaller is better, S-type, is
$$ \large\displaystyle MSD=\frac{{{\left( {{Y}_{1}} \right)}^{2}}+{{\left( {{Y}_{2}} \right)}^{2}}+\cdots +{{\left( {{Y}_{n}} \right)}^{2}}}{n}$$
The formula for nominal is better, N-type, is
$$ \large\displaystyle MSD=\frac{{{\left( {{Y}_{1}}-{{Y}_{0}} \right)}^{2}}+{{\left( {{Y}_{2}}-{{Y}_{0}} \right)}^{2}}+\cdots +{{\left( {{Y}_{n}}-{{Y}_{0}} \right)}^{2}}}{n}$$
In this example, n = 1 as we did not replicate the experiment. Thus, we only have a single Y value for each run.
This simplifies the equation to
$$ \large\displaystyle MSD=\frac{1}{{{Y}^{2}}}$$
Factor |
level | ΣY | Y | MSD | S/N |
A | A1 | 35 + 25 | 30 | 0.000625 | |
A2 | 39 + 27 | 33 | 0.001322 | ||
total | 63 | ||||
B |
B1 | 35 + 39 | 37 | 0.000816 | |
B2 | 25 + 27 | 26 |
0.000946 |
||
total | 63 | ||||
C | C1 | 35 + 27 | 31 |
0.000609 |
|
C2 | 25 + 39 | 32 | 0.001371 | ||
total | 63 |
The signal to noise values and final analysis
The MSD is a stepping stone to calculating the signal to noise ratio, S/N.
The different MSD formula permits a common analysis for any situation by comparing S/N values. A higher S/N value indicates a stronger influence on the response.
Depending on the experimental objective that means a higher S/N may indicate a higher, lower, or nominal influence on the response.
S/N is calculated using the MSD with this formula
$$ \large\displaystyle S/N=-10log\left( MSD \right)$$
Running out the calculations of S/N for our example experiment, we find
Factor |
level | ΣY | Y | MSD | S/N |
A | A1 | 35 + 25 | 30 | 0.000625 |
32.04 |
A2 | 39 + 27 | 33 | 0.001322 | 28.79 | |
total | 63 | ||||
B |
B1 | 35 + 39 | 37 | 0.000816 | 30.88 |
B2 | 25 + 27 | 26 | 0.000946 |
30.23 |
|
total | 63 | ||||
C | C1 | 35 + 27 | 31 | 0.000609 |
32.15 |
C2 | 25 + 39 | 32 | 0.001371 | 28.63 | |
total | 63 |
It is the magnitude of the S/N difference that tells the story.
The larger the difference between S/N for each factor’s levels, the more that factor influences the results. Think of each factor a tuning knob, the larger the difference in S/N the more control or range of responses that factor exhibits on the results.
If there is little difference, as with factor B, then there is little difference in response for either level selected.
In this example, factor C has a difference of about 3.5 and factor A has a difference of about 3.3.
A difference of 3 db (the units of the signal to noise ratio) are significant. A difference of less than 3 db does not mean there is not a difference between the factors, it is just not enough convincing data to see the difference clearly.
In this experiment, in order to maximize the conversions, we should set factor A and C to level 1.
The level for factor B has no clear winner, so you could set level 1 or 2, whichever helps you meet your constraints (such as cost).
When no other considerations suggest a level, select the higher S/N value, so in this case, we would select factor B’s level 1.
The result of the analysis suggests that run 1, all levels set at 1, will maximize the conversions. The result suggests one of the four runs had the correct configuration.
This is not always the case, therefore complete the analysis before implementing the solution.
Thank you so much for this post. I’ve been studying Experimental Design with Applications in Managament, Engineering, and the Sciences. They explain everything about Taguchi methods EXCEPT how to analyze them.
But, now that I try to recreate your MSD from the results, no matter how I whack it, I don’t get what you get. Instead of:
.000625
…
I get:
(1/35)^2 + (1/25)^2 (all divided by one) for -> 0.00241
1/30^2 for -> 0.0011
…
I really, really don’t know how you got your number. Please help!
Hi Aaron, thanks for the notes and I’ll have to get back to you one the calculations. I am traveling and limited time, etc… Cheers,
Fred
What does this column represent: ΣY Also how did you calculate these values?
i agree with Aaron, I don’t get what you get for the MSD column.
It’s simple because mad values which are mentioned in table are based on the top table y values.
I think he mistakely did.
Beautiful explanation of results are given by him.
It plays main role in my master’s project.
Thanks Fred.