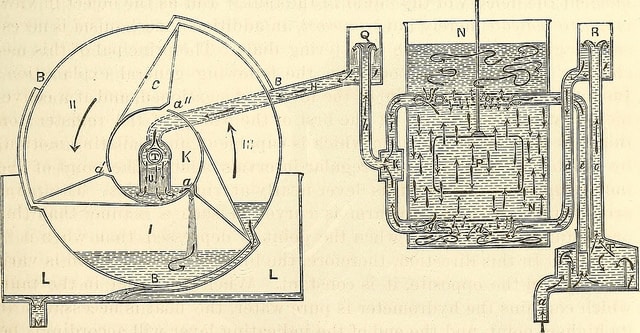
The MTBF Conspiracy Theory
When my son was young he asked a lot of questions that were difficult to answer. For example:
- Why is the sky blue?
- Why do I have to go to school?
- What is a conspiracy theory?
The first two were expected, yet the third set me back a little. How do you explain conspiracy theory to a 5th grader? The dictionary type definitions just seemed to confuse everyone. So, I made up a conspiracy theory.
I said, “Did you know, North Dakota, is not really a state?”
For those that haven’t heard of North Dakota, which on many maps is in the north central part of the US, that just reinforces the theory that it doesn’t exist.
My son, having recently memorized all fifty US states and their capital cities in school, said I was wrong and he even knew that was true as he still recalled the capital city name.
“Prove it.”, Was all I said in response.
“Well it’s on the map on the country as a state.” My reply included how maps change and are arbitrary. Anyone could have drawn the map, and how do we know it is accurate. Maybe the good folks in South Dakota paid the map maker to draw in the fictions state of North Dakota.
“It’s listed in Wikipedia!” And, my reply, was about how anyone can create a posting on the site, what is the proof it’s actually true? Have you ever seen a car with ND plates or meet someone from there?” He hadn’t.
My son knew I was only demonstrating the idea of a conspiracy theory. We had fun with it for years.
I was glad he never asked me,
“Why do people use MTBF?”
Just with the blue sky, a shrug and smile just wasn’t a good enough answer. There has to be a rational reasons people use MTBF.
After writing about perils of MTBF use for a few years, my current theory is it has to be a conspiracy.
The MTBF conspiracy theory revealed
Here’s what I think happened.
A bright engineer was tasked with estimating the reliability of a nuclear submarine’s electronics. He was given about a month to achieve this task, which is not enough time to conduct any testing. So, he gathered all the component failure rate data, tallied it up and reported the expected failure rate. {Parts count prediction}
The marketing department noticed the failure rate value and the word failure. The admission that the submarine might fail didn’t help to sell summaries, so they flipped the failure over, creating the average time between failure, or mean time between failures, MTBF.
The lower the failure rate the higher the MTBF went. Up was good. Failure is bad. {That’s how I think marketing folks think – sorry}
The engineers understood failure rates the math to create MTBF was pretty simple. So whatever, tis the same thing. Then management got involved.
The management team only wanted to read and talk about MTBF {again the word ‘failure ’ is bad thinking}. They set MTBF goals, they expected glowing reports of increasing MTBF values, and so on.
Then something really bad happened.
The US Military created a standard. And, a company used a computer to automate the standard’s estimate of MTBF. Other’s did too. Now there was profit to be made by estimating MTBF, not reliability. So, they sold MTBF estimations. After all, that is what the management team wants, MTBF.
The military standard spawned many industry standards. The standards become parts of purchase contracts. MTBF flourished.
“What is your MTBF?” became an acceptable way to ask about reliability performance.
The murky bit of the theory involves why very few stood up to say, “Let’s not use MTBF, it is not very useful. Let’s use the probability of success over a duration (reliability) instead.” You may have said these very words or words to the same affect. And you felt the resistance.
- We always use MTBF.
- Everyone in our industry uses MTBF.
- The vendor only provides MTBF values.
My theory is we all know better, {maybe not the marketing folks – sorry} and we just do feel able to overcome the resistance to change. We know we could do much better with better metrics, yet the backlash is unrelenting.
Just as that first engineer figured out a quick way to come up with a failure rate estimate, we too face the necessity to use MTBF. We do not have the time or energy to change our company or industry to stop using MTBF. So, we just do it.
It’s easy.
I don’t know if the spread of MTBF use is organized by a secret group or not. I suspect not. Yet the ease of use and avoidance of the word failure (or anything the smells like we would have to do statistics) conspired to trap us into using MTBF.
That’s my theory. If you know of any critical bits of information to support this theory, let me know. If we expose the conspiracy for what it is, it may just fade away. We then may get back to work doing reliability engineering and creating reliable products.