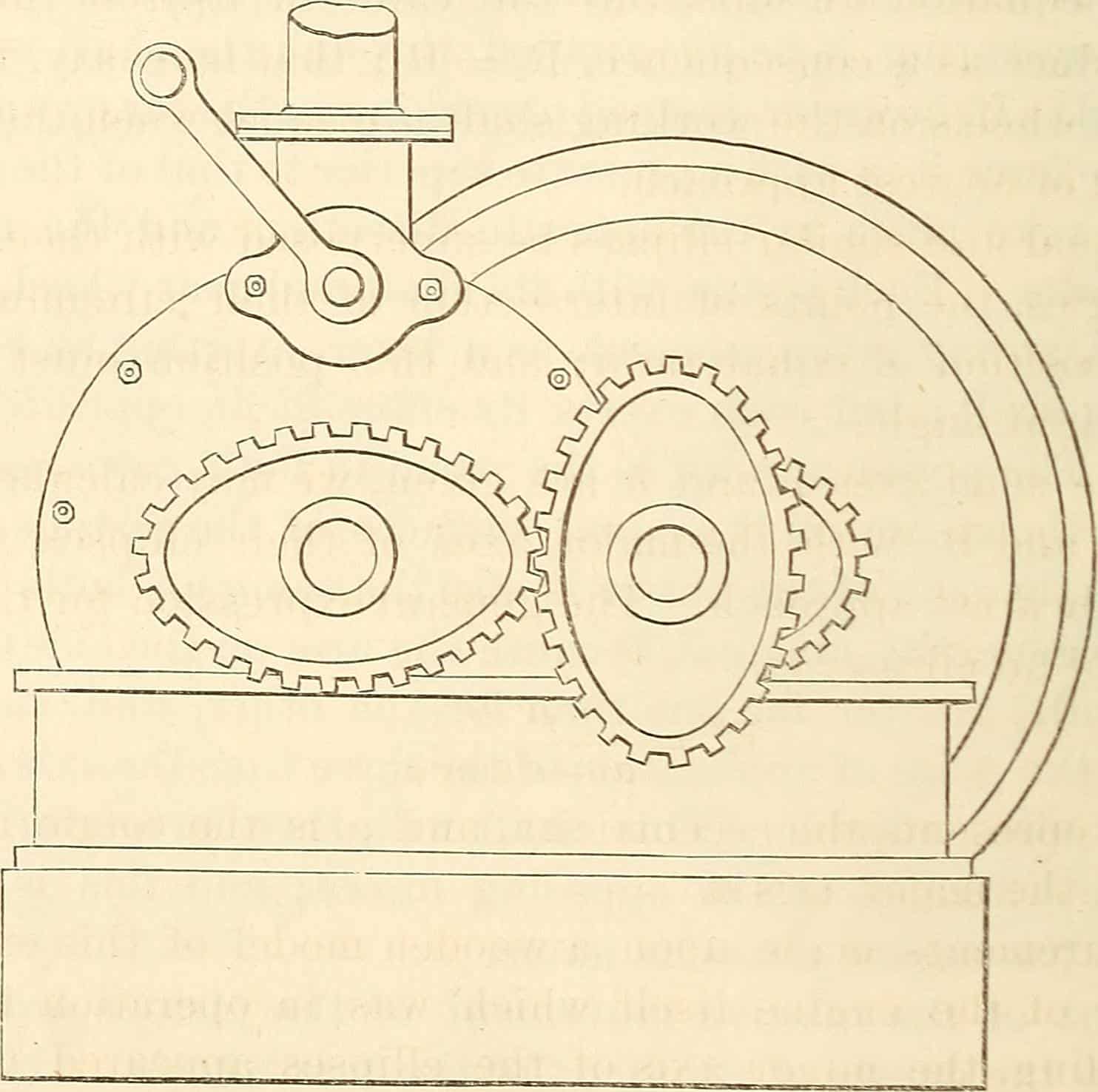
Considerations When You Calculate MTBF
It is deceptively easy to calculate MTBF given a count of failure and an estimate of operating hours. Just tally up the total hours the various systems operate and divide by the number of failures. Easy.
This simple calculation is the unbiased estimator for the inverse of the parameter lambda for the exponential distribution, or directly to estimate theta (MTBF). We use theta to represent the 1 / lambda.
What could go wrong with such a simple calculation?
What is a failure?
Let’s start with what we count or do not count as a failure. This directly changes the resulting MTBF value. If we only count confirmed hardware failures, and do not count intermittent or unreproducible or software failures, are we under counting what the customer experiences as a failure?
Over what duration do we count the failures? Should we focus only on the first month of operation, the first year, the warranty or service contract period or the entire operating life of the system? How do you calculate MTBF?
Some organizations only count failures they expect to occur. The unexpected ones are ‘special’ causes and require further study before counting as failure officially.
Another organization only counted failures that completely shut down the system. A partial loss of functionality, a degradation of capability or the failure of a redundant element all did not count a system failure.
In my opinion if the customer calls it a failure, it’s a failure. If a failure, by any definition, costs your organization time and money to address, acknowledge, resolve or repair, it’s a failure.
What is operating time?
This one is tricky. If the system does include the appropriate sensors and tracking mechanisms (hour meter) and a way to gather that operating time of units both failed and still operating, then we have a pretty good way to track total operating hours. Some situations and systems make this easy.
Most do not.
Let’s say we ship 100 systems a month for 10 months. At the end of ten months the first shipments have accumulated 10 months of operating time. IF….
… They are all placed into service immediately
… They are all operated full time for the full 10 months
… They are have each failure reported including down time
In general, we do have to make a few assumptions to determine the operating time for shipped systems. We tend to be conservative and err on the side that would make the MTBF value a little smaller than if we had the full set of carefully tracked data. Or do we?
- Some organization count from date/time of shipment ignoring shipping and installation time.
- Some organization assume all systems are installed and operated 24/7.
- Some organization assume no news is good news and the systems with no information are still operating.
And a few organization assume systems run indefinitely, even systems 20 years old, unless notified that it is decommissioned, assume it is still running full tilt. i.e. No retirement or replacement policy.
How about when you calculate MTBF?
By convention when there are no failures we assume in the next instant there will be one failure. This avoid dividing by zero which causes fits for calculators and spreadsheets and mathematicians.
Another issue is how often are the calculations made? Do we gather data hourly, daily, weekly, monthly, annually? Some use a rolling set of data, for example only units shipped in the last year count for both operating time and failures. This result will ignore or discount the longer term wear out failures as the bulk of the units are young.
Some organization do the calculations weekly in order to detect trends. If there are trends you probably should not be using MTBF…. If it’s changing, if there are early life or wear out failure mechanisms, you should not be using MTBF.
Even though you can calculate MTBF easily, the complexities of getting it right still do not provide a useful metric. Instead focus on getting better data including time to failure information so you can explore and report the data with other tools and methods. Treat the data appropriately and make better decisions
Sure, better data will improve the ability to calculate the MTBF value, if you’d like to be like some organizations, that is fine.
How have you seen MTBF calculated poorly? Share your thoughts and stories in the comments below.
Related:
Perils of using MTBF