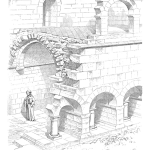
What are the covariances of Kaplan-Meier reliability estimates at different ages? I need them for the variance of actuarial demand forecasts and for confidence bands on reliability. I thought cohort reliability estimate variances and covariances in the previous article were a good idea. How good? Not as good as bootstrap and jackknife resampling alternatives!
The Kaplan-Meier reliability function estimator uses right-censored and grouped time-to-failure counts in periodic cohorts (rows in table 1). The Nelson-Aalen cumulative failure rate function estimators are theoretically independent [Aalen, Nelson], but not for some examples. The Kaplan-Meier reliability and actuarial failure rate function estimates at different ages are dependent, so their covariances matter to actuarial forecasts and confidence bands on reliability.
Bootstrap, Jackknife, or Empirical Covariances?
Maximum likelihood estimators’ variance-covariance matrix converges asymptotically to the Cramer-Rao lower bound. The Cramer-Rao bound is for unbiased estimators. The Kaplan-Meier estimator is biased, so real data may not be close to asymptotic and the variance and covariance the Kaplan-Meier estimator may not be close to the Cramer-Rao bounds. Greenwood’s variance formula is an estimator of the variance of the Kaplan-Meier reliability estimate, a Cramer-Rao lower bound on variance [Freedman, Greenwood].
The previous article compared the Greenwood standard deviations with empirical cohort reliability estimates’ standard deviations for the data in table 1 [George]. Empirical cohort reliability estimates are: R(0)=1; R(t)=R(t-1)*(1-d(t)/(n-∑d(s)); s=1,2,…,t-1, where d(t) is cohort deaths at age t and n is cohort size. Cohorts are independent, although cohorts have different sizes, different numbers of grouped failure counts, and different maximum ages (aka Type 1 censored). Averages of the empirical cohort reliability estimates are unbiased. Multiple cohort reliability estimates provide data for estimation of the reliability variance-covariance matrix.
Table 1. Ships, grouped failure counts [“Weibull Analysis of Perplexing Field Data,” by James McLinn] and Kaplan-Meier reliability estimates
Week | Ships | 1 | 2 | 3 | 4 | 5 | 6 | 7 | 8 | 9 | KMRel |
1 | 20 | 1 | 0 | 1 | 0 | 1 | 0 | 1 | 0 | 1 | 0.9882 |
2 | 50 | 1 | 0 | 1 | 0 | 1 | 0 | 1 | 0 | 0.9882 | |
3 | 70 | 1 | 0 | 1 | 0 | 1 | 0 | 1 | 0.9746 | ||
4 | 100 | 1 | 0 | 1 | 0 | 1 | 0 | 0.9746 | |||
5 | 100 | 1 | 0 | 1 | 0 | 1 | 0.9578 | ||||
6 | 100 | 1 | 0 | 1 | 0 | 0.9578 | |||||
7 | 120 | 1 | 0 | 1 | 0.9278 | ||||||
8 | 120 | 1 | 0 | 0.9278 | |||||||
9 | 146.07 | 1 | 0.893 |
Bootstrap resampling from the Kaplan-Meier estimate (“KMRel”) makes data for estimation of the variance-covariance matrix of reliability function estimates at pairs of different ages [https://en.wikipedia.org/wiki/Bootstrapping_(statistics)/]. (Condition on reliability between 0.893 and 1.0.)
Jackknife sampling computes multiple Kaplan-Meier reliability estimates from all-but-one cohorts (leave one out) [https://en.wikipedia.org/wiki/Jackknife_resampling/]. The jackknife makes data for estimation of the variance-covariance matrix. In this example, the jackknife only generates eight reliability function estimates. The bootstrap generates as many as you want.
Reliability function standard deviation estimates increase with age (figure 1), because there are fewer cohorts with data for older ages. The Greenwood formula under-estimates the standard deviations, because it is an asymptotic bound for unbiased reliability estimators. Empirical cohort reliability standard deviations (“Emp Stdev”) over-estimate standard deviations, because they are for different estimator than the Kaplan-Meier.
Tables 2 and 3 show alternative variance-covariance matrix estimates. Notice that the jackknife gives mostly negative covariances? The jackknife computes means and standard deviations from dependent subsets! That makes me suspicious of jackknife covariances.
Table 2. Bootstrap variance-covariance matrix of the Kaplan-Meier estimator. Covariances are non-negative and not negligible.
Age, Week | 1 | 2 | 3 | 4 | 5 | 6 | 7 | 8 |
1 | 8.7E-05 | 9.3E-03 | ||||||
2 | 8.7E-05 | 8.7E-05 | 9.3E-03 | |||||
3 | 2.6E-05 | 2.6E-05 | 8.2E-05 | |||||
4 | 2.6E-05 | 2.6E-05 | 8.2E-05 | 8.2E-05 | ||||
5 | 4.3E-05 | 4.3E-05 | 7.3E-05 | 7.3E-05 | 1.4E-04 | |||
6 | 4.3E-05 | 4.3E-05 | 7.3E-05 | 7.3E-05 | 1.4E-04 | 1.4E-04 | ||
7 | 7.2E-05 | 7.2E-05 | 5.8E-05 | 5.8E-05 | 1.4E-04 | 1.4E-04 | 2.6E-04 | |
8 | 7.2E-05 | 7.2E-05 | 5.8E-05 | 5.8E-05 | 1.4E-04 | 1.4E-04 | 2.6E-04 | 2.6E-04 |
Table 3. Jackknife variance-covariance matrix of the Kaplan-Meier estimator. Most jackknife covariances have opposite signs from bootstrap covariances!
Age, Week | 1 | 2 | 3 | 4 | 5 | 6 | 7 | 8 |
1 | 1.7E-05 | |||||||
2 | 1.1E-05 | 8.4E-06 | ||||||
3 | 3.6E-06 | 8.0E-06 | 3.6E-05 | |||||
4 | -4.1E-05 | -2.2E-05 | 2.2E-05 | 1.6E-04 | ||||
5 | -5.6E-05 | -3.6E-05 | -8.1E-07 | 1.6E-04 | 1.6E-04 | |||
6 | -6.8E-05 | -4.5E-05 | -1.1E-05 | 1.8E-04 | 1.8E-04 | 2.9E-04 | ||
7 | -4.0E-05 | -2.2E-05 | 1.8E-05 | 1.5E-04 | 1.5E-04 | 1.8E-04 | 1.4E-04 | |
8 | -5.5E-05 | -3.5E-05 | -1.6E-06 | 1.6E-04 | 1.6E-04 | 2.2E-04 | 1.4E-04 | 1.9E-04 |
Averages of empirical cohort reliability function estimates differ from Kaplan-Meier reliability function estimates, but they are unbiased estimators. The variance of the average cohort reliability at age 1 is VAR[(d(1,1)/n(1)+d(2,1)/n(2))/2], and the variance of the Kaplan-Meier reliability at age 1 is VAR[(d(1,1)+d(2,1))/(n(1)+n(2))], where d(I,j) counts deaths at age j from cohort I of size n(i). So, bootstrap the Kaplan-Meier estimator for its variance-covariance matrix, if you do not want the variance of the empirical cohort reliability functions.
Recommendations and Questions?
Greenwood variance estimates may be wrong depending on data. Covariance estimates differ depending on method. Check alternatives: empirical cohort, bootstrap, and jackknife reliabilities and their covariances. The Jackknife mean is theoretically unbiased but its covariances are suspicious because of dependence and limited number of jackknife subsamples. Compare variances of actuarial forecasts computed from alternative variance-covariance matrix estimates.
Empirical cohort reliability estimates are computed from independent cohorts, unlike the jackknife. Although cohort durations differ, empirical cohort estimates’ variances, and covariances should be more precise than jackknife estimates.
Should I use weighted variance-covariance estimates [Khan et al.] to compensate for different size cohorts? Should I derive weights that minimize distance of the empirical variance-matrix from the Cramer-Rao bound? Others impute the actual lives of censored observations [Vinzamuri et al.] to avoid using the Kaplan-Meier estimator.
You may ask, “How will you estimate the variance-covariance of nonparametric reliability estimators from ships and returns counts, without grouped failure counts? The sample reliability function covariance may not be close to the Cramer-Rao bound. Send field reliability ships and returns counts data and I will show you: pstlarry@yahoo.com.
References
Aalen, O. O., “Nonparametric Inference for a Family of Counting Processes,” Annals of Statistics, Vol. 6, 701726, 1978
D. A. Freedman, “Greenwood’s Formula,” https://www.stat.berkeley.edu/~freedman/greenwd.pdf
Larry George, “Variance of Kaplan-Meier Reliability?” Weekly Update, https://lucas-accendo-site-speed.sprod01.rmkr.net/variance-of-the-kaplan-meier-estimator/#more-508828/, March 2023
Greenwood, M., “The natural duration of cancer. Reports on Public Health and Medical Subjects,” Vol. 33, pp. 1–26, His Majesty’s Stationery Office, London, 1926
Habib Nawaz Khan, Qamruz Zaman, Fatima Azmi, , Gulap Shahzada, and Mihajlo Jakovljevic, “Methods for Improving the Variance Estimator of the Kaplan–Meier Survival Function, When There Is No, Moderate and Heavy Censoring-Applied in Oncological Datasets,” Frontiers in Public Health, May 2022
James McLinn, “Weibull Analysis of Perplexing Field Data,” ARSymposium, 2010
Wayne Nelson, “Theory and Applications of Hazard Plotting for Censored Failure Data,” Technometrics, Vol. 42, No. 1, February 2000
Bhanukiran Vinzamuri, Yan Li, and Chandan K. Reddy, “Calibrated Survival Analysis using Regularized Inverse Covariance Estimation for Right Censored Data,” IEEE Transactions on Knowledge and Data Engineering, DOI:10.1109/TKDE.2017.2719028, June 2007
Why not compute the variance of broom chart Kaplan-Meier reliability estimates? Jerry Ackaret told me that’s what he called my graphs Sequent HDD of K-M reliability estimates of successively larger cohorts sizes and grouped failure counts. Shucks, I can’t paste a broom chart here. Anyway, I can post the K-M broom and Greenwood standard deviations. Greenwood underestimates standard deviation! This uses the data from Jim McLinn.
K-M Greenwood
0.0056 0.0036
0.0056 0.0036
0.0161 0.0059
0.0076 0.0059
0.0185 0.0088
0.0082 0.0088
0.0189 0.0152
0.0060 0.0152
Beware the Greenwood variance! It is a lower bound, for unbiased estimators, asymptotically, which means as age->infinity and reliability=>0.