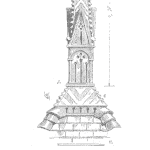
“How many samples do we need?” is a very common question. It is one you will receive when planning nearly any kind of reliability testing. It is a great question.
Having too few samples means the results are likely not useful to make a decision. Too many samples improve the results, yet does add unnecessary costs. Getting the right sample size is an exercise starting in statistics and ending with a balance of constraints.
There are six elements to consider when estimating sample size. We will use the success testing formula, a life test with no planned failures, to outline the necessary considerations.
Statistical Considerations
The variance of the population is rarely known when contemplating sample sizes. It is important to know the value or to at least have a reasonable estimate. The more variability, larger the variance, the more samples you will need for any sample size.
When the population has a larger amount of variability, the ability to detect or measure a statistic (mean, standard deviation, etc.) accurately becomes more difficult than from a population with a smaller variance.
The population variance is what it is unless you first take on a project to minimize and stabilize the population variability. This is often not possible.
Generally, we use a sample size to measure something in order to answer a question.
- Is this design better than another?
- Does vendor A have a longer life then vendor B’s solution?
- Will this product last at least 5 years with fewer than 2% failures?
The ability to discriminate or detect changes is an element of sample size calculations. The ability to detect a small difference requires more samples then spotting a large difference.
Finally, the confidence value impacts the sample size requirements. If we want to take very little risk that the sample represents the population we will require more samples, then if we are will to take more risk (lower confidence). Confidence here relates to the chance the sample comes from one end or the other of the population range of values thus distorting the representation of the population based on the sample.
Business Considerations
Cost is a consideration, of course. Samples cost money. Even inexpensive samples take time to gather, plus they take resources to handle and measure. Cost is the most common business consideration.
One way to judge the cost of a sample set is in comparison to the value of the information the sample will provide. If the performance improvement based on selecting the right vendor’s parts is worth 10x the cost of the samples and testing, then it is likely worth it. Of course, a 10x return may or may not be sufficient within your organization.
A related consideration is the importance of the decision being made based on the sample. If it is a $10 decision (where to have lunch today, for example) you may not need any samples, just go to lunch. If the decision is a $768,000 decision, then gathering enough samples to make the best decision is well worth it (if the samples and testing costs are sufficiently low.
Another consideration is the purpose of the sample. If conducting an exploratory set of experiments to learn how a design responds to different stresses, then one or two samples may provide sufficient results.
Whereas a complex accelerated life test that provides evidence behind durability claims to customers, then taking the appropriate steps to use sufficient samples is in order.
Success Testing Sample Size Formula
The success test approach attempt to replicate the set of stresses or use of a product over its entire lifetime. For example, if a car door hinge will experience 10,000 open/close cycles over its lifetime, then we may design a test to simply open/close some set of car doors 10k times each.
The following formula is from the Binomial distribution when zero failures occur. The sample size, n, set of doors in this case, if they each cycle without failure for 10k times each (m is the lifetime, which is 1 lifetime in this example), then we can claim the reliability, RL, has been demonstrated with confidence, C.
$$ \displaystyle\large n=\frac{\ln \left( 1-C \right)}{m\ln \left( {{R}_{L}} \right)}$$
Two of the three statistical considerations have representation in this formula. C is the confidence. A common value is 0.9 for a 90% confidence.
The RL is the reliability value, in our example let’s say we want to demonstrate at least a 90% reliability over the lifetime of 10,000 open/close cycles.
The discrimination consideration is not within this formula as it is a one-sided experiment looking to demonstrate some minimum reliability value. This is a binomial-based experiment with either a sample has a failure during the cycling or not. In other testing that includes some measurement which has variability than would require a term to address the discrimination desired.
This means if all the samples cycle over one lifetime (here 10k cycles) then we have a 90% confidence that the population has at least a 90% reliability. If we wanted to demonstrate a higher reliability value and/or with high confidence we would require more samples.
Summary
As a statistician, more samples are better, yet calculation how many samples you need based on the statistic of the experiment is the first step. Then consider and balance the sample size with the appropriate business considerations.
We rarely get enough samples for all that we want to do. Consider the consequences of too few samples as obtaining results that are not useful for any decision making. Then focus on using samples for those critical or important decisions. Spreading too few samples over too many experiments will likely result in little useful information and many poor decisions.
Use statistics to estimate sample size requirements then balance risk, cost, and other factors to find the right sample size for your particular experiment.
A pretty good formula for a quick estimation of sample size. So completing the math from the car door example to demonstrate a Reliability of 90% with a confidence of 90% we need 22 car doors and each must cycle 10k times with zero fails. I’ve never really used the “m” since the lifetime is already accounted for in the Reliability goal over the usage life. Also as a note an easier way to remember the formula: R^n = 1 – CL
Then just solve for “n” by taking the natural log of each side:
ln(R)*n = ln(1-CL)
n = ln(1-CL) / ln (R)
Thanks for completing the math Sam, and 22 is the sample size if the test runs for one lifetime with 90% reliability and demonstrating at least a 90% reliability.
the m or number of lifetimes is pretty handy if, in this case, we could run for 20k door open/close cycles, then m would equal 2, meaning we would only need 11 samples, if none failed, to demonstrate 90/90.
Cheers,
Fred
Hi Fred,
Do you have a reference for this formula (including the m)? I agree, I’ve never seen the m included.
Would be
Max
One place I’ve seen this formula including the m is in Gary’s book, in chapter 6 Test Sample Size Determination under section 6.4.2 Extended Bogey Testing Under Exponential Life Model – sections following include m with Weibull sample size formulas as well.
Wasserman, Gary S. Reliability Verification, Testing and Analysis in Engineering Design. New York: Marcel Dekker, 2003.
cheers,
Fred