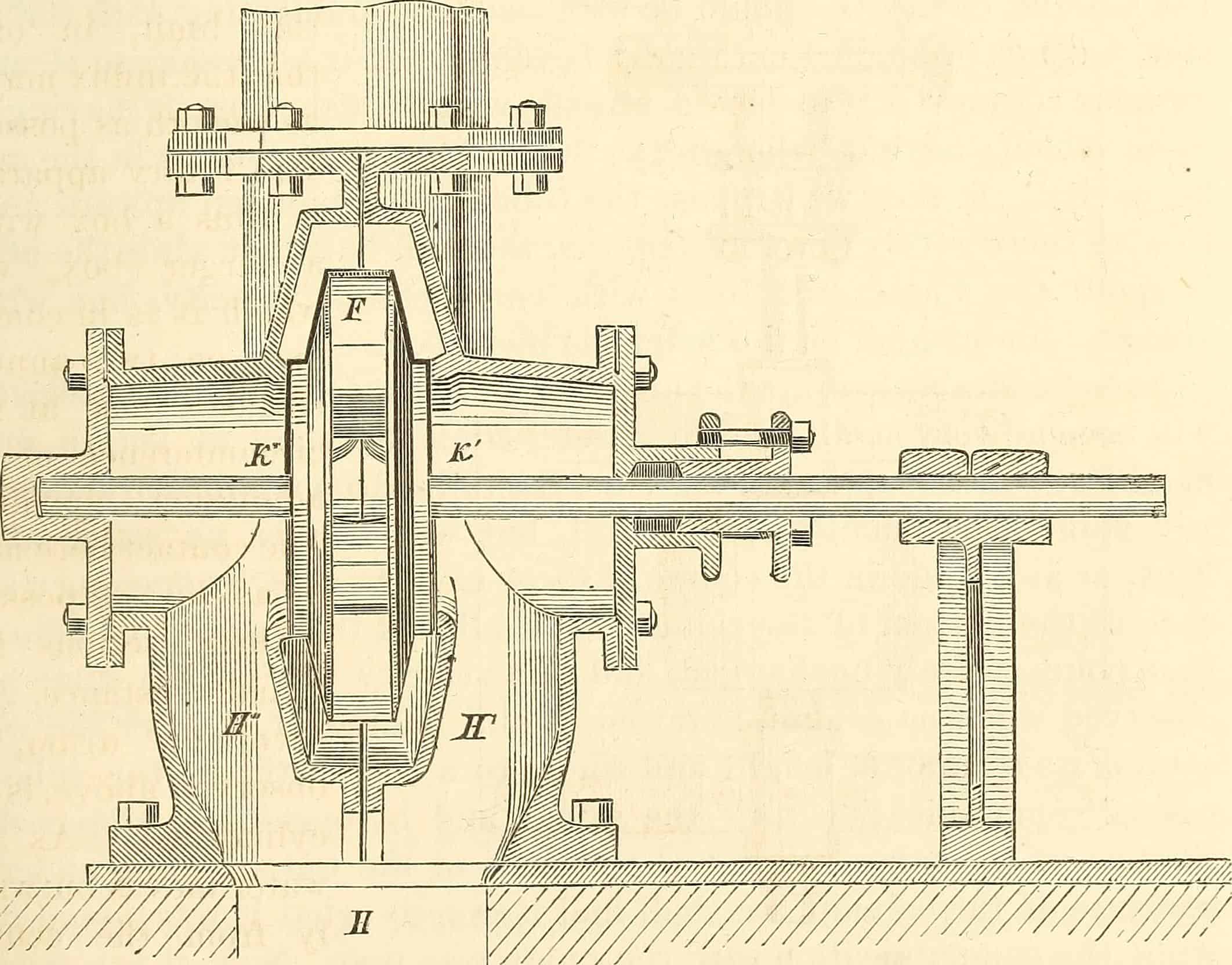
Do You Have Enough Data?
To make informed decisions you need information.
To form conclusions you need evidence and a touch of logic.
To discover patterns you need data.
In each case, and others, we often start with data. The data we have on hand, or can quickly gather.
We organize data into tables, summaries into reports, display in dashboards, and analyze the results to form decisions.
It is possible to summarize a million data points with a single number. Is that useful or meaningful? Probably not unless it is just the right bit of information needed to form a decision. You could also display the 1 million data points in a complex multidimensional plot complete with interactivity, color coding, and other fancy options. Is that necessary even if it is possible?
Awash in Data
In many organizations the data is every where. There are terabytes of storage units brimming with sales, product configurations, call center records, and field returns. In your factory you have machine repair records, sensor trips and resets, plus product measurement data. You most likely have a lot of data collected for one business reason or another.
Is your data useful for you as a reliability engineer?
Just because you have a lot of data doesn’t necessarily mean it is useful. You may have the number of field returns last month, yet missing when the units were put into service, the month or week of production, and likely also missing the root cause of each failure.
Just because you have a lot data about your equipment and maintenance activities, you likely are missing when the motor was placed into service, how many hour it operated since the last servicing check, plus likely also missing the root cause of each failure.
Do have a record showing the engine was checked along with the oil replaced? Did the check include an oil analysis looking for metal or continents? Do you have a record of a customer product return simply described as “doesn’t work”? You probably have a lot of data, yet not enough of the right data.
Getting the Right Data
This morning as the sun broke the horizon it back lite some wisteria blooms in my yard. The light changed the color and intensity of the flowers. I took notice of the flowers in their new environment.
Getting the right data often takes shining the light on the results and conclusions that are possible given the right data.
With field returns, that root cause may not be in the data, so get your hands on a few returns and conduct root cause analysis. Do this a few times and highlight how this data provides a necessary element to all the other data to help you and your organization make informed decisions.
With the next oil change, secure a sample of the old oil and send it out for analysis. The insights will supplement your existing data to help you and your organization form better conclusions.
If you do not know how long something has been in service, guess or use left censoring data analysis. Highlight your assumptions and why you need better data. If you need to know the month of production, find someone with access to the right database and get the data (explain how the analysis will directly help them with their job, too).
When your team is making a decision about a design or new equipment, cost may be an important element. That data is readily available. How about the impact of a change if failure rate on the cost of ownership (warranty) or operation? Does the team making the decision have the failure rate data and cost of a failure information? Sometimes we have to provide the right data at the right time, too.
Show how using the existing data along with the right data help you and your organization improve results, conclusions and decisions. Doing so helps the bottom line and your career.
One of the issue we’ve faced during the process of generating life data analysis from fielded data, is the lack of of data for each “usage profile” we might have 10 similar assets from the same manufacturer with the same model, but each used for different reasons of different parameters in different environment which introducing unique failure modes for each usage profile.
Hi Ammar, very true and not often available. We are seeing more equipment with sensors and logging capability to monitor the environment, hours of operation, etc. Often we have to make the business case for the data and the benefits to both the end user and suppling organization can certainly help the case. cheers, Fred
We typically know the month/year a product shipped, and when it came back failed, but only get the operating hours on about 10% of returns. Question is how long did it actually operate, and how long from time it shipped until it went into service. Actually got a reasonable representation in many cases by linear regression of service hrs. vs. months since shipped for the ones we did have data on, and showed both hrs. / month as slope and months between ship and entry into service (like a Weibull gamma) as the intercept. Your data might not be clean enough as far as usage for this to work, but if it is, it gives you a way to fill in the blanks on your population, both failed and suspended units in service.
Thanks for the note Kevin – you describe a very common situation – and yes often the data is noisy. cheers, Fred