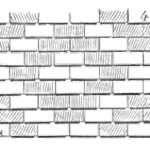
Customers experience product failures.
Understanding these failures that occur in the hands of customers is an essential undertaking. We need this information to identify increasing failure rates, component batch or assembly errors, or design mistakes.
Our work to design for reliability includes assumptions about customer expectations and use stresses.
The field performance either validates our work or illuminates the errors. Our work to select suppliers and build a stable assembly processes attempts to identify the highest risk elements for reliability (and quality) with plenty of assumptions. The field performance again validates our work or illuminates the errors.
The field reliability performance impacts the business directly. Customer satisfaction, brand loyalty, and warranty expenses. The business objectives hinge of reliability performance.
Customers may expect product improvements even if they did not experience the failures personally. The internet provides many venues for customers to compare notes and discuss failures.
Customers increasingly do not simply want a replacement they may demand an improved design instead.
The Nature of Field Data
This data is never perfect.
It is better than anything we create in the lab though.
Field data is actual data.
It is a record of how the product performs for those using the product. All the expectations, stresses, and component variation are present. No sample sizes or confidence bounds necessary.
Part of the issue is we the data from customers is noisy. We do not know exactly when the first turn on or use occurs after purchase. Nor do we know exactly and under what circumstances failure occurs. Often, we do not know the exact failure. Just that a customer reports a failure. Not all customers even provide a complaint or report.
Yet it is the best data we have available.
Find the Field Data
Your organization most likely gathers information about customer experienced field failures. Call centers, return authorizations, replacements, repairs, warranty claims, all provide information on field failures.
Ideally, you will have date installed, date of failure, use conditions, symptoms or failure mode, plus a root causes analysis of each failure to the specific mechanism. Right. More likely we have the date the customer reported the failure, which may not be the same as when it actually failed.
When first looking for the field data, it is often gathered for other purposes.
The databases and records are to help serve the customer and track costs, not to reveal reliability performance. As you and the organization realize the value of the field data analysis, you’ll be able to establish better data capture processes.
Another element of data you require for an analysis is the number of units placed in service, both those that have failed and those that have not failed. The shipments data is often a good source. Better would be records of initialization or turn on.
This may be complicated by delays in shipping and warehousing. Or with the use of units as spares.
Likewise, not all units installed and operated continue to operate indefinitely. This may take some work and investigation to determine the nominal and range of operating durations.
Most simply assume every unit shipped is still operating unless reported as failed.
Gather the Field Data
A common mistake is to simply count the number of returns each month and report the count on a month by month bar chart.
This is easy and generally non-informative. Trends are likely to be caused by variation in shipments as any other reason.
Trends are likely to be caused by variation in shipments as any other reason.
Beyond how many failures occurring do you need to gather the time to failure information as a minimum?
When was it shipped, installed, failed and reported would be great, yet knowing the month of shipment and month of failure is often the best we can do.
The time to failure data allows Weibull analysis or similar to estimate the overall failure rate trend versus the age of the product. Time zero is when installing (or shipped) for each unit. Do they show signs of wear out (increasing failure rate) after 3 months?
The conditions of use and reported failure mode provide a way to Pareto the issues.
Adding the cost to the customer or the manufacturer may provide a way to refine the priorities for improvement work.
Plot the various failure modes or better failure mechanisms with the Weibull analysis as each one is likely to be on a different failure rate trend. Some will indicate early life failures and other may show wear out behavior.
At different points in the age of the product, the Pareto of issues is likely to be different.
What happened?
The last but often most useful element of the field data is finding out what happened.
Do the root cause analysis on as many units as possible. Determine the sequence of events or stresses that lead to the product failure. If it’s not possible to redesign or improve the current product, you can on the next design cycle.
We’ll explore how to analyze the data in another article, yet gathering the data is often the difficult part of the exercise.
Do you have good data? Where do you find is the best source of field data?
Related:
Field Data and Reliability (article)
Field Data Analysis First Look (article)
The Next Step in Your Data Analysis (article)
For shame!
Life data is sufficient but not necessary to make nonparametric estimates of age-specific field reliability. Ships and returns counts are statistically sufficient, and they’re required by GAAP.
Sure those estimates from ships and returns counts are in calendar time and include sell-through time, and perhaps exclude lagged reporting of recent failures. It’s easy to deconvolve sell-through time and detect lagged reporting. Some operations take place in calendar time, but, if somebody wants reliability in operating hours, then convert using the distribution op-hours per calendar hour. I have used distribution of field time of DoAs
Is it worth time, cost, and trouble of tracking everything by name and serial number from birth to death? What is the cost of uncertainty due to using only ships and returns counts? Answer lies in lies in Kullback-Leibler divergence and Kelly betting optimization. If anybody is interested, send field reliability data, lives or counts, repairable or not, and I will send back nonparametric estimates of field reliability and failure rate functions, asymptotic variance-covariance, and quantify costs of uncertainty.
thanks for the note Larry – and for those interested in take Larry up on his offer to analyze your data, do so. He’s a good guy and will provide meaningful information back to you.
Larry, would you be willing to draft an article for posting here on how you analyze the ships/returns data and how or why that is better/worse then using a basic Weibull fitting based analysis?
Cheers,
Fred
Thanks for the rational response to my tirade. I am working on comparisons of reliability estimates with vs. without life data, “Random-Tandem Queues and Reliability Estimation, without Life data,” (including repairable systems).
My offer to estimate reliablity from ships and returns counts still stands.
I’ve already written about nonparametric estimation of reliability and failure rate functions from ships and returns counts: http://sites.google.com/site/fieldreliability has a couple of articles, and there are more, depending on the applications.
For example, a long-time disbeliever and skeptic finally sent 5 years of monthly ships and returns counts. So I sent him back nonparametric reliability estimates and broom charts that showed infant mortality and reliability (not MTBF a la Duane, Crow, AMSAA, et al.) growth. The estimates also showed a bit of return rate around age one year and a teensy bit or wearout Then he told me the product was not used for more than two years, so I revised the estimators to take that into account, and the results did change, but still showed infant mortality, one-year glitch and teensy bit of wearout. Then he told me warranty was a year, so I revised the estimates again, but not much difference (except for wearout just before two-years of age).
How are you going to see what really happens if you assume Weibull? OTOH maybe people would like https://sites.google.com/site/fieldreliability/weibull “How to Make Data Fit Weibull?” Would you like examples of not-Weibull? See references to that article for a couple. I am looking for more. Got any?
I forgot. I actually have a max. likelihood Weibull reliability estimation workbook somewhere around here. Its inputs are ships and returns counts.
And I have my original Apple computer workbook circa 1990 for max. likelihood Weibull estimation of Terry Baiko’s rolling reliability life data. It was on the Internet until Comcast yanked my web site.
Hi Fred,
I have a small doubt over here, Can we generalize that Reliability value of a product that we get after ALT testing will in most cases be greater than that of field reliability? and here to be more specific by a product I mean RPD’s (Respiratory Protective Devices).
If yes can you please help me with a suitable article or some Research paper.
Thanks in advance.
Hi Sai,
Keep in mind that accelerated life tests typically focus on one failure mechanism and when a product is in the field many different failure mechanisms may cause a product’s failure. ALT tends to explore wear out types of failures, while in the field we may also have early life failures… so yes, in general, the field failure rates for the entire product or system will typically be at a higher rate then from the results of ALTs.
Cheers,
Fred
Good question. It’s a testable hypothesis:
Ho: ALT reliability function = field reliability function for reasonable ages vs.
Ha: Not equal for at least some reasonable ages
1. If you have life data, let me know if you want “Product Reliability Comparison with Censored Data,” or “To the Man With a Hammer, Everything Looks Like a Nail,” ASQ Reliability Review, Vol. 17, No. 1, March 1997
2. If you don’t have field life data, send ships and returns counts. GAAP requires that data but you may have to work to get it.
Error 404 Link not found. It is at end of article among related articles.
https://lucas-accendo-site-speed.sprod01.rmkr.net/2015/12/03/field-data-reliability/
Thanks Larry, got the links fixed. cheers, Fred