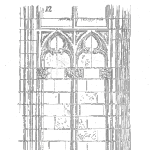
The tasks of a Reliability Engineer are long & diverse. While heavily dependent on the industry one is working in, it generally involves all aspects of the Equipment – from Design to Manufacturing to Operation to Maintenance. Even though the responsibility is wide, the resources available for a Reliability Engineer within an organization are limited. Often, there are only a few Reliability Engineers managing hundreds of Equipment. Given this current situation, the arrival of AI seems like a perfect resource to complement the work.
The technological aspect of AI that will be a great fit here is Machine Learning (ML). It is the concept where patterns are learned from the input data without explicit programming. It opens new ways to analyze data that were previously deemed difficult to perform computations. Six common applications of ML in Reliability Engineering are given in this reference link.
Let’s explore some common responsibilities and how AI complements Reliability Engineers:
Reliability Engineer’s Task | Done without AI | Done with AI |
Equipment Performance Monitoring | Manually comb through data trends from Automation systems, Warranty database, CMMS, etc. | AI monitors the performance & Alerts user of performance deviation |
Equipment Reliability Prediction | Make Life Cycle predictions (Weibull Analysis) at regular intervals of the program | Automated analysis predicts Reliability % at any given time |
Root Cause Failure Analysis | Conduct investigation, collect associated data, assess failed component, and discuss with team to determine Root Cause | Diagnostic algorithms will detect data changes & highlights the contributing parameter to Failure |
Data Collection | Data from different systems: CMMS Workorders, Field Warranty Reports, Testing Reports need to be combined for analysis | Test extraction from reports (pdf, images), multiple data sources combined to create Training dataset, Data Pre-processing methods |
Design Optimization | Design drafted, built, and iterated after testing. Iteration is linear. | Optimization algorithm finds the best design layout given program inputs & constraints |
The table above shows that certain sections of the tasks are executed best by an algorithm. They are precise, accurate, reliable, and faster in processing data of high dimension & size. But it is important to note that the algorithm will not replace the Reliability Engineer. It is a perfect companion to them just like a calculator or an analysis software, but the final interpretation of the results and decision-making must be done by an experienced Reliability Engineer.
This is not an exhaustive list. New algorithms are being researched and new applications are explored by industry & academia. One of the lesser appreciated tasks of Reliability Engineer is to communicate the findings to other teams and Management. Can we adapt ChatGPT, MS CoPilot, Vimeo One-Take to make persuasive presentations & reports? Yes, we can.
The only real limitation of complementing AI in Reliability Engineering work seems to be in the imagination!
Leave a Reply