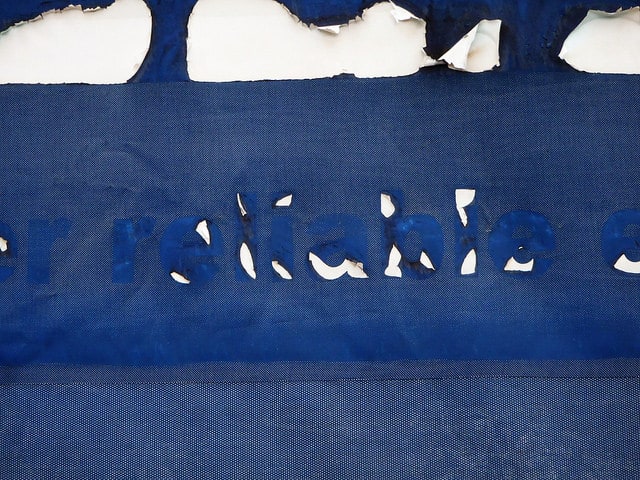
How to Estimate MTBF

Every now and then I receive an interesting question from a connection, colleague or friend. The questions that make me think or they discussion may be of value to you, I write a blog post.
In this case, there are a couple of interesting points to consider. Hopefully you are not facing a similar question.
The Question
Recently received a message that asked how to improve the estimated MTBF using Mil Hdbk 217 parts count prediction. They had input the parts, filling in missing information based on vendor data, included the correct operating temperature, derating, etc. The result was less then half the desired goal.
What do do?
My Response
First, stop using Mil Hdbk 217, it is not meant to provide estimates of reliability or MTBF, it’s outdated, uses very bad assumptions, etc. Just stop.
Second, there are many ways to torture the data to provide pretty much what ever MTBF value you want. Don’t you’d be stretching very poor practices over a faulty method.
Third, given parts count prediction methods are not much different than random number generators, use the low value to encourage the team to improve the system reliability (fewer parts, lower power consumption, lower operating temperature, etc.).
Basically, don’t use parts count and don’t worry about the results. It’s not worth your time to get a different number. Nor worth our team’s consideration or use with any decision making.
A Few Thoughts
Why won’t 217 just go away? Maybe it’s the power of no copyright and the document being just about everywhere. Also, it’s been in use for so long, in some way it has become associated with reliability predictions despite not intended to do so by the authors.
When you see someone trying to use 217 – help them avoid the folly of their ways.
What would have happened if the initial result was good enough? How would the result been used? Most likely to confirm the assumptions that the new design was reliable enough. That may have been the sum total of reliability work for the program. Sad.
217 and other part count models are useful for comparisons, for what if analysis, and similar studies. Like what happens if we add a cooling fan or increase the derating across the power supply. 217 is not very good at prediction reliability performance.
The premise of the question raised a concerned with the number not meeting expectations. Interesting that the response was to adjust the prediction to meet the expectations instead of increase the reliability of the design to meet goal. As a reliability professional we understand that no model or estimate will fully predict the customer actual experience. Some methods are better than others, yet a pure fiction generating method no matter how adjusted or tweaked will be useful in the long run. We really should be working to identify risks and helping the team design a robust and reliable product.
Maybe the product is very robust and reliable and the customer requires the 217 prediction as a condition of purchase. In this case, the low value may influence the sale unnecessarily. The parts count prediction is only being done to satisfy a customer request. That could happen.
Given this circumstance, do you craft a 217 based report that doesn’t cause too many questions safe with the knowledge the real performance will meet their needs. Or should you provide both the 217 based report plus the much more informative data (may a system level Weibull plot of the expected life distribution)? Take the opportunity to educate your customer? You may have to educate your sales team first.
Methods to Estimate MTBF
Parts count methods are to be avoided to estimate field performance – just don’t use these methods.
Field data analyst – use similar products field results to estimate the reliability over time. Best using a life distribution or model that related time to chance of failure, yet even a count of hour of operation divided by number of failures provides a simple estimate of MTBF.
Life testing and modeling – use the data you have available to populate a reliability block diagram. For new or critical items conduct life testing and update the model. Use the model to estimate the number of failures over time for a MTBF estimate.
Physics of failure modeling – when not data exists and you either do not have time or resources for life testing, use existing stress/failure models to create an estimate of reliability performance.
Or just make something up, let everyone know it is just a random number, and go about doing real reliability engineering work instead of creating MTBF estimates.
What are your thoughts on adjusting a 217 prediction to meet expectations? How do you estimate reliability (please don’t say you have to estimate MTBF…. )
Help question MTBF
WILDER