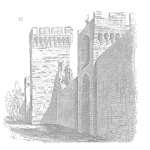
We make decisions all the time. Often our decision making is with little more than a gut feeling.
When faced with a major decision we often look data to help us decide. Is the product reliable enough as designed? Which field returns indicate we should stop production?
Some decision may help us earn or lose thousands if not millions of dollars.
Deciding to delay a product launch by six months means we have no revenue for the duration. The delay may also permit us to address a design flaw that would cause half the products to fail within a few months.
The later may cause loss of market share, erosion of brand loyalty, not to mention the cost of warranty claims.
Data and statistics
Data is a pile of numbers.
Readings, observations, or measurements generate data. The data will likely show variability. Not every prototype has the same voltage reading at test point 28.
Often we make measurements or run experiments on development prototypes or demonstration units to gather data. The data we can use to evaluate or compare the performance to a criteria or standard. Some experiments seek optimization while others investigate strength limits (found at point of failure). These measurements vary.
The key is the data on a set of samples does not provide the same values as if we measured all production units, yet we often use the prototype samples to project conclusions about all future production.
We are using a sample set of readings to create statistics which are estimates of population parameters.
The key term here is ‘estimate’
For example, when we measure the length of five units, we have five measurements or pieces of data. If we calculate the mean and standard deviation of those five measurements, we have two statistics, the sample mean and sample standard deviation.
These two sample values are an estimate of the universe of production samples yet to be constructed. We are going to base our ‘ready to start production’ decision, in part, on the sample data and the resulting statistics.
The tabulation of data dates back to the Egyptians. Eves, Howard W. “A Very Brief History of Statistics.” The College Mathematics Journal 33, no. 4 (2002): 306-08. They likely didn’t use hypothesis testing or confidence intervals as those were invented later.
Today we can use modern tools to fully understand the data we have collected to make informed decisions.
Comparisons and decisions
If we have five readings of length we have a limited ability to make a decision.
We often compare the readings or their statistics to something. A specification, for example.
Are the five-length measurements within the length specification? If so, is that sufficient to conclude all units will meet the specification?
Probably not.
We know or recall from college stats courses, that the sample has a chance of not representing the population. The samples may have all been ‘good’ or ‘poor’ units in their ability to describe the measurements one would receive across all units.
A cluster of five readings tightly groups near one of the specification limits may single a problem or not. It may mean close to having the unit will fall outside the specification or none will.
We need a bit more analysis to determine which is the true case (and more samples wouldn’t hurt, either)
Our ability to make comparisons based on data alone is limited. We need to understand the variability of the data. We need to use statistics to help understand what a sample or any set of data is actually revealing.
For decision making, we can use statistics to manage the risk the sample statistics are close to the true and unknown actual population values.
This allows us to estimate the risk of making a wrong decision or the right decision.
Measurement error and decisions
Every measurement, reading or observation recorded has an element of error.
The offset between the actual length of a product and our measured length is measurement error. Part of the variability of any reading is the measurement error, which is variable. If we measure an item 10 times with an accurate enough gauge, we will get ten different readings.
Measurement error alone can determine if a product is good or not. If actual and unknown true length is just inside the upper specification limit, that is good, yet can well tell?
The measurement of length will include a bit of error. It may be less than the actual and unknown value resulting in the decision the length meet specification and is good.
Or, the error may result in a value outside the specification. The conclusion then is the length is too long and we decide the part is outside the specification thus bad.
We use statistics to evaluate the capability of our measurement systems, thus enabling us to improve the capabilities or at least understand the measurement error impact on the readings.
Reliability and statistics
When evaluating if a device design meets reliability objectives we may employ a life test.
Other options include accelerated life testing, field data analysis of similar devices, or existing models relating stress/environment conditions to time to failure estimates.
In each case, we employ statistics to gather and analyze the original data. Regression and goodness of fit methods are statistics in action. Optimization or part acceptance testing likewise use statistics.
We are looking for the probability of failure based on the data we collect. We are using the data to estimate time to failure and frequency of failure type information.
Not every unit is the same. Not all use conditions and frequency of use are the same. It is the variability of the unit to unit variation and environmental and use condition variability that leads to the time to failure variation.
Not all units will survive exactly 100 years and a day as the One Hoss Shay. Time to failure varies, thus we use statistics.
We use statistics to describe time to failure distributions, or plan demonstration testing, or when comparing our life estimates to reliability objectives.
We must, (or at least we should), use statistics to make decisions.
Related
Show me the data (article)
Basic Approach to Achieve Process Stability (article)
How to Encourage the Use of Statistics (article)
Making Use of Reliability Statistics (podcast)
Leave a Reply