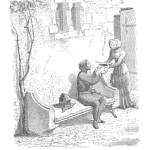
Similar to the Weibull distribution yet with slightly heavier tails. While not as easy to interpret if the data shows early life or wear out features, the lognormal distribution often fits time to repair data accurately.
Transform the data by taking the natural log of each data point. The resulting values tend to be normally distributed if the original data fits a lognormal distribution.
You can use base 10 or base 2 or any base and the results will still tend to be normally distributed. It is common to use natural log, ln().
According to the central limit theorem, as we sum random variables, as the sample size increases, the distribution of the sum become a normal distribution.
The individual data may have any distribution. Using the ln transformation and the central limit theorem, as we multiple lognormal random variables, as the sample size increases, the distribution of the product become a lognormal distribution. Again given any distribution of the individual items.
Recall that the ln of the product of random variables equals the same of the logarithms of the individuals.
$$ \large\displaystyle \begin{array}{l}y={{x}_{1}}{{x}_{2}}{{x}_{3}}\\\ln \left( y \right)=\ln \left( {{x}_{1}} \right)+\ln \left( {{x}_{2}} \right)+\ln \left( {{x}_{3}} \right)\end{array}$$
Probability density function, PDF
The PDF for the lognormal distribution is
$$ \large\displaystyle f\left( x \right)=\frac{1}{x\sigma \sqrt{2\pi }}{{e}^{-\tfrac{1}{2}{{\left( \tfrac{\ln \left( x \right)-\mu }{\sigma } \right)}^{2}}}},x>0$$
Where, μ is the location parameter or the ln mean
And, σ is the scale parameter or ln standard deviation.
The location parameter, μ, is the mean of the transformed data, likewise the scale parameter, σ, is the standard deviation of the transformed data.
The lognormal distribution starts at zero and runs to positive infinite thus is skewed right. Depending on the value of the standard deviation the distribution may appear similar to the exponentials distribution or the normal distribution.
Cumulative density function, CDF
The CDF for the lognormal distribution is
$$ \large\displaystyle F\left( x \right)=\Phi \left( \frac{\ln \left( x \right)-\mu }{\sigma } \right),x>0;\sigma >0.$$
Where Φ(x) is the standard normal cumulative distribution function.
Thus the lognormal distribution exhibits many of the benefits for analysis as the normal distribution.
Related:
Calculating Lognormal Distribution Parameters (article)
Central Limit Theorem (article)
The Normal Distribution (article)
Fred,
The lognormal model can only represent wear out failure modes (always skewed right). The Weibull can represent all failure modes, so the similarity between the two is very limited.
Mark Powell
If you have a point-coordinate on the CDF and the scale parameter (or ln standard deviation), how do you calculate the location parameter (or ln mean)?
I have got two questions concering working with lognormal distributed data;
1. How can you calculate μ and σ when you only have got the mean and the standard deviation of the raw data?
2. If you analyze the normal distribution by logtransforming your data, do you need to retransform the outcome in order to get accurate concentrations for instance?
Hi G
thanks for the questions.
First, if all you have is the mean and std dev of the raw data – you have what you need to define the lognormal distribuiton. Some books use the log of the data then calculate the mean and std dev, while others use the raw data mean and std dev directly as the lognormal parameters. Basically, one uses the mean of the log data then the other use the log of data mean – both get to the same place.
What you lose without having the raw data is the ability to determine if the lognormal accurately describes the raw data.
The second question – keep in mind that the normal distribution ranges from negative infinity to positive infinity. the log normal is only defined from zero to positive infinity. The two distributions really define different patterns for data, one that plus/minus and the other only plus side of the number line.
If the data is well defined by the normal – use that, it has plenty of benefits. If the data is better described using log normal, then use that.
cheers,
Fred