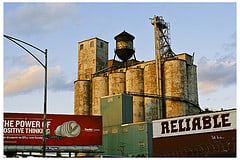
Does MTBF have any role in Maintenance?
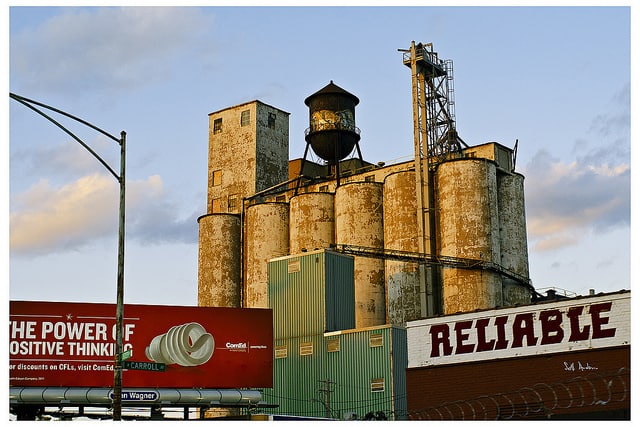
No. You should not use MTBF when designing or scheduling maintenance programs or tasks. Furthermore, it is a very poor metric to monitor equipment performance.
The basic calculation of MTBF (or MTTF) and assuming the equipment time-to-failure distribution is the exponential distribution implies the equipment downing event occurs randomly. In other word the equipment doesn’t break in and actually lower it’s chance for failure over time, nor exhibit wear out or the increase of failure rates over time.
The chance of failure is constant over time and does not change given the time the system or component has been in service.
MTBF dose provide the average time between failures and does not provide any information about when the failures may occur if the actually failure do not occur randomly. Furthermore the exponential distribution has a memoryless feature, meaning a motor that is brand new and a similar motor with1,000,000 hours of service each have the same chance to fail in the next hour.
The MTBF calculation or vendor supplied value does not include information about how the failure rate may change over time.
Wear Out and Maintenance Planning
Let’s use a motor as an example for a simple maintenance planning exercise. Let’s say the motor has an MTBF of 100,000 hours provided by the vendor. There isn’t any maintenance on the motor, such as lubrication or alignment checks, yet we are planning to use 100 motors in the plant and need to plan for spares.
How many spares will we need over the next year to replace faulty motors.
Using just MTBF, we can use the probability of successful operation over the year, 8760 hours, and quickly estimate how many of the 100 motors will require replacement.
$latex \displaystyle&s=3 R(t)={{e}^{{-t}/{\theta }\;}}$
t is 8760 hours
θ is the MTBF or 100,000 hours
Thus, we find 91.6% of units should survive one year of operation. That means out of 100 installed motors, we expect about 8.4% to fail, or 8 or 9 units. Of course we could add a confidence bound to this calculation plus include the time the replacement unit operate for a bit more accuracy. For this example we’ll keep it simple.
Yet, we know based on experience with other similar motors that they rarely fail during the first year. With a little work we find the motors do actually wear out primarily due to bearing wear. And another call to the vendor we find they recommend using the Weibull distribution with β of 2 and η of 90,000 hours.
The reliability function for the Weibull distribution is
$latex \displaystyle&s=3 R\left( t \right)={{e}^{-{{\left( {}^{t}\!\!\diagup\!\!{}_{\eta }\; \right)}^{\beta }}}}$
Where η is the characteristic life, in this case 90,000 hours
And, β is 2.
Thus over one year we would expect 99% of the motors to survive, meaning only 1 is expected to fail.
Using MTBF would have us buy 7 or 8 extra spares unnecessarily.
Maintenance Scheduling
We know that motors wear out. Given only MTBF and the exponential distribution assumption we do not have sufficient information to schedule motor replacements.
If the motors actually failed randomly, as assumed, then our only strategy is to replace motors as they fail. Since the chance to fail each hour remains constant arbitrarily replacing motors at a any point in time will not avert or change the chance of failure the next hour.
When we model the wear out behavior, I.e. Weibull distribution with β of 2, then we can calculate the time at which the chance of failure is economically unacceptable. For example, if we typically operation in 1 week shifts of 168 hours then have time for maintenance tasks, we can calculate the chance of failure over a week period after one year, two years, etc. And determine when the chance of failure becomes unacceptable.
Knowing how the failure rate changes over time we can schedule replacements and maintain a relatively lower overall failure rate.
Summary
Find or estimate the information concerning the changing rate of failure over time. Ignoring wear out or early failures by using MTBF only will cost you and your plant money.
Understanding and modeling the wear out patterns allows you to secure spares as needed. You can avoid costly downtime by doing replacements before the chance of failure is too high.
PS: I’m working on examples and update to the draft book on MTBF to include more maintenance reliability specifics.
Hi Fred
A very good article—more or less i agree.
One question, in the article you have examined reliability data from the motor vendor
Weibull distribution with β of 2 and η of 90,000 hours.
If we do not want to use only MTBF/ MTTF values. Do you have experience in other “Weibull distribution “, not only on motors but also on contactors, relays etc. What about a powersupply with capacitors, they are also exposed to “wearout”
What would be “the best guess”
Per
Hi Per,
Thanks for the note. The Weibull distribution for the motors in the articles is just an example. Not real data. For any component if you have time to failure information fit the data to a Weibull and find your parameters.
http://www.barringer1.com has a listing of beta and eta ranges for many different types of parts. Some have very large ranges, like motors, due to the many types of motors and use conditions.
I typically fit data to a Weibull distribution as it is so versatile. Lot’s of experience with Weibull and with many parts and systems.
Cheers,
Fred
Hi Fred,
Just to avoid the confusion, you may like to change in your article “The reliability function for the Weibull distribution” as
\displaystyle R(t)={{e}^{-{{\left( {t}/{\eta }\; \right)}^{beta}}}}
thanks, you’re right, really should include the function.
Cheers,
Fred
Hi Fred,
Again the assumptions on Beta and Eta.
“I typically fit data to a Weibull distribution as it is so versatile. Lot’s of experience with Weibull and with many parts and systems.”
You do need failures (and a good analyse of them) to get a Weibull distribution, even more, you need failures with the same root cause.
If you have different root causes you have different Weibull distributions.
For a maintenance phase you probably would expect the Beta and Eta values from the development tests (if they have record of that).
Hi Tim,
I learned that the first step when confronted with a stack of data is to plot it. A histogram, a probability plot, stem-and-leaf, what ever kind of plot and often do many plots. What I have found with time to failure data, of any form or structure or source, just plotting it helps you form good questions that lead you to a proper analysis.
I highly recommend plotting the data – Weibull is informative as a starting point, which is why I recommend it.
Sure, life is great if all the failures are due to one failure mechanism, sure there are still plenty of assumptions and issues. Yet, we do need to move forward with an analysis and I’ve found asking insightful questions about the data is a great start.
Might have to do a blog post on using Weibull for different types of analysis, or case studies, etc. Want to join me and provide some examples of how you analyze field data?
Cheers,
Fred
Hi Fred
This question still haunts me.
Why Weibull is applicable for only one failure mode. Is it an assumption of carrying out this analysis?
What would happen to Weibull distribution if we consider different failure modes altogether.
For example: If we plot Weibull distribution for a pump having seal failure, bearing failure, coupling failure etc.
Hi Shitiz,
I do this regularly. For a product like the pump and various elements that fail – plotting the pump time to failure, and each failure mechanisms provides a nice chart showing the different individual failure plus the system failure. I’m assuming any failure causes the system to fail and after the repair the system is as good as new.
For repairable systems it is also informative to use the mean cumulative function plot. See http://nomtbf.com/2012/02/graphical-analysis-of-repair-data/ for details. It is a non parametric plot that provide useful insights for repairable systems.
Try a few different plots and take a look, ask good questions and improve the product, system or process – that’s the goal, not perfect analysis.
Cheers,
Fred
Thanks Fred.
I like your last statement. It really makes sense.
Hi Fred,
Would love to see more posts on the Weibull analyses.
We should get it as widely spread as possible I think.
I is just that I see Weibull distributions used to calculate reliability without any clue why the Beta and Eta used is chosen.
You actually cannot assume a Beta and Eta without prior knowledge of a similar product or failure type.
Kind Regards,
Tim
Hi Tim,
I’ll work on it – always looking for topics that have some interest 😉
And, if you’d like to write up a few articles that would be wonderful. I know you know this stuff.
Cheers,
Fred
Hello Fred,
Nice article.
I´m trying to do such calculation for a product, which had its MTBF and Failure Rate calculated based on the electronic components on the board.
It has 10 years warranty, but I can´t say the characteristic life.. How can I use the Weibull distribution with these data to predict the amount of spare parts?
Thanks Thiago for the kind words. If all you have is MTBF and Failure Rate, likely from a parts count prediction, you really do not sufficient information to estimate spares. You essentially have a fictional number that does not relate to actual field reliability performance.
Even is the estimated MTBF is accurate, it suggests that the only appropriate maintenance strategy is to replace upon failure. So, if I were you, I’d get a few spares and track actual data until you can properly determine the appropriate spare stocking level.
Cheers,
Fred
Hi Fred- this is an item that I am currently speaking with the developers of OPUS 10 about. They have responded to my query by stating that it doesn’t matter what distribution is used, their tool’s results don’t differ much- for systems in steady state.
The critical part of their response is the mention of steady state. Most systems I deal with never get to steady state, and inj my industry, the use of OPUS 10 is mandated in many projects. I’ve seen the results first hand, by being in touch with projects over long time frames. As an example, circuit breakers. The OEMs often cite ridiculous MTBFs, but given a “reasonable MTBF of , say, 180k hours, versus a Weibull char life of 160k hours and beta of 2, a 10 year sparing shows a demand of 3 for the exponential, and 1 for the Weibull. For time frames beyond 20 years, the average demand is similar, but for periods less than the true mtbf, the results are terrible. Whilst I think MTBF is bad in many ways, I don’t subscribe to “noMTBF” as you do. Actually knowing what MTBF is part of providing that expertise as a RE.
Hi Tim,
Good luck with the software folks – as you mention the evidence that we don’t achieve steady state alters the results.
I agree REs need to understand MTBF and educate others that MTBF is not Reliability and often not useful for making any type of decision. Then provide alternatives that are useful.
Cheers,
Fred
PS: thanks for reading the articles and commenting, much appreciated