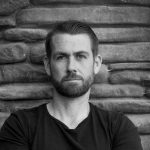
Rational Assumptions
Abstract
Chris and Fred discuss the roles assumption play in reliability. Assumptions can be very useful … or very dangerous. Why? Because assumptions are information. If they are based on nothing, then assumptions become misinformation. Which means you make the wrong decision. Listen to this podcast to learn more!
Key Points
Join Chris and Fred as they discuss the role assumptions play in (reliability) engineering. Why are assumptions so prevalent? Because assumptions are information. So if you have no idea about something, making an assumption essentially throws information at your problem. Which is great! If the assumption is valid. And if it is not? Then you have misinformation.
Topics include:
- Assumptions can be great! In fact, most statistical analysis requires you to assume a model that describes the data you are seeing. If you are really making a ‘guess’ … then always go back and validate your assumption. This is really easy …
- What happens if you get it wrong? You are essentially making decisions for ‘Product X’ based on characteristics of some – potentially fictitious – ‘Product Y.’ Which is dangerous.
- WeiBayes … what is this? The way it is used today … really bad. A Weibull distribution has two parameters and is often assumed to model failure data. Bayesian analysis involves bringing prior, ‘non-test’ information to your analysis to improve the outputs. And what is WeiBayes? Simply assuming one of the parameters for your Weibull distribution is a fixed value. Again … this is an assumption. And those of you who know what Bayesian analysis is truly about, know that this is a dangerous oversimplification of that approach.
- Assumptions don’t change the underlying physics and chemistry of failure. They can make our life simpler. There was once a (so-called) reliability engineer who tried 15 different models to see which one meant they needed the fewest samples for testing. And then they assumed that model described the time to failure of their product. Flat out ridiculous.
- But don’t be afraid to make assumptions if you have the information. Let’s say that you are looking at a new bearing. Well … not a new bearing. The same bearing with a different lubricant. You don’t have a lot of bearings to test. So perhaps you are going to assume the shape parameter that describes the nature of the time to failure of the ‘old’ bearings won’t change. And this is often a valid assumption. ‘Shape’ parameters tend to describe the nature of failure, while ‘scale’ parameters describe the typical or characteristic time it takes for your bearing to fail. Making this assumption can reduce the number of bearings you need to test. And if you really want to do Bayesian analysis properly, you can put what we call a prior distribution into your model which describes the likely values of the shape parameter. This is smart.
- Do you assume models and distributions to make your life easier – or because they are based on evidence?
Enjoy an episode of Speaking of Reliability. Where you can join friends as they discuss reliability topics. Join us as we discuss topics ranging from design for reliability techniques to field data analysis approaches.
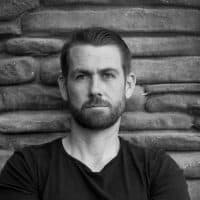
- Social:
- Link:
- Embed:
Show Notes
Leave a Reply