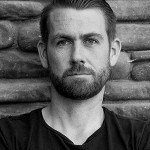
Interpreting Distribution Parameters
Abstract
Chris and Fred discuss what ‘distribution parameters’ mean when it comes to random processes. Specifically failure random processes. This is an interesting podcast in response to a question from one of our listeners – which are podcasts we love!
Key Points
Join Chris and Fred as they discuss a question directed to us by a listener. In fact they were two questions – as follows:
Think of probability distributions and the sequence you define your observation points. Neither the distribution type nor the parameters change e.g. when you reverse the sequence or change the order. It’s ambiguous to me, because if I have higher rate of failures in the past but better conditions now, I’d like to see it in my parameters and shape. Otherwise, how can I rely on e.g. beta in my Weibull distribution? 2) How may I determine the rate of events (say rate of TTR, TTF, or any other parameter) when my distribution is not Weibull? Which parameter should I use? Let me appreciate your time & willingness to help in advance. Keyvan.
Just for the uninitiated, a Weibull distribution is a type of probability distribution that is used a lot in reliability engineering. The ‘beta’ refers to what we call a shape parameter, which describes the nature in which failure occurs.
Topics include:
- Order of data shouldn’t matter … if we are looking at time to failure. The first step of any random data analysis is to order the data from smallest to largest. Unless … we are talking about a ‘renewal process.’ This is where you might have a single machine that works until it fails, and then it is repaired, and it keeps working. In which case … the order of data does matter. In a renewal process, one machine might have lots of times to failure (noting it gets repaired). This means that we can’t use probability distributions to describe single times to failure (like a Weibull distribution).
- But what if it is a renewal process? Then we can perhaps examine monthly failure rates, or the Mean Cumulative Function (MCF) to identify trends over time. And by trends, we mean failure rate behaviors that show wear-in, wear-out or something in between. If you are really interested in getting to the bottom of what is going on, then research this thing called the nonhomogenous Poisson process.
- OK … so what if it is simple ‘time to failure?’ Well before we talk about ‘betas,’ we need to confirm the Weibull distribution is an appropriate model. It is sometimes useful to break down failure into failure modes. If there are different failure modes, they might be modelled by different Weibull distributions. For example, if a system fails due to wear-in around half the time, and wear-out the other half, then fitting a Weibull distribution might try and ‘average’ the two and (incorrectly) conclude the system has a constant or non-changing failure rates. So always confirm you have the right model.
Enjoy an episode of Speaking of Reliability. Where you can join friends as they discuss reliability topics. Join us as we discuss topics ranging from design for reliability techniques to field data analysis approaches.
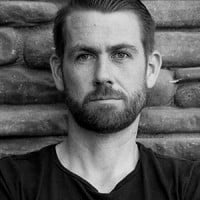
- Social:
- Link:
- Embed:
Show Notes
Hello Fred & Chris,
First and foremost, thank you so much for this interesting conversation over the questions I posted earlier 🙂
I understood I should have explained the context better. I do it now! I work mainly in the continuous processes (dominantly in the Oil & Gas). Thus my case studies are mainly repairable mechanical or electrical equipment items with few failure data points over a course of years. I am trying to promote using RAM as a supplementary solution to less quantitative solutions such as RCM, RCA, RBI, etc. We do try to improve equipment / system bad actors using identification of failure modes and then mechanisms.
Now, listening to this episode, I developed further questions:
1) Somewhere in the show, Fred speaks about Weibull being not applicable for repairable items. I wonder if this is right when you can repair your equipment “as good as new”.
2) Chris speaks about renewal process & NHPP. I would love to hear about both of them more (characteristics, applications).
3) Recommending using instantaneous hazard rate to represent changes in the failure rate sounds like a great solution. Yet, couple of questions:
– Do you know how to formulate it in Excel?
– Fred promotes using instantaneous hazard function h(t). Why not using a cumulative hazard function H(t) instead, just the way the CDF was promoted to be used?
4) Recommending to cluster the datasets before drawing the distribution was critical. So far I was thinking both Weibull & Crow-AMSAA are strong distributions when it comes into “dirty data” (ref: Abernethy, R., 2006. The new Weibull handbook). I understand now that I had a wrong perception of it. Would be nice if you run a show also with respect to Crow-AMSAA and it’s applications especially in production / process reliability. For instance, is it wrong if I put all my datasets into one pot, calculate beta of my Crow-AMSAA and conclude if the system reliability is deteriorating, flat or improving over time?
5) I don’t get the exact difference between MTTF & MTBF. I understand they refer to non-repairable and repairable items respectively, but when it comes into calculations and my items can be repaired as good as new, how do they differ from each other?
I certainly do not expect you to cover all these questions, but perhaps you can address few of them on the upcoming shows. My feeling is that you have had quite a number of episodes regarding HALT but very few with topics like the above ones.
Thank you for these interesting episodes and keep up the good work.
BR-Keyvan
Hi Keyvan,
thanks for the listen and additional question. These certainly will work for future topics.
In the meantime – question 1) if the repair is to good as new and the same element fails the say way each time, then the Weibull is suitable as it is based on on failure mechanism and time starts at zero after every repair. This is rarely the case hence the advise to avoid using time to failure distraibuiton to analyze repaired system data.
The rest I’ll leave to Chris to address or as topics for future shows.
cheers,
Fred