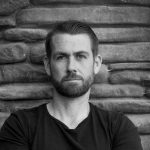
Practical Way to Learn Statistics
Abstract
Chris and Fred discuss statistics! Yay! And how we can be better at using them in a practical way. And that starts with wanting to learn about statistics. But how do we make that happen?
Key Points
Join Chris and Fred as they discuss statistics and how we can better use them in a practical way. Statistics are often seen as a concept to avoid at all costs. Or pretend to know without really knowing. As they say … there are lies, damn lies and statistics. So what can we do to have statistics help us in a more meaningful way?
Topics include:
- Make statistics relatable. Many ‘learning’ institutions start statistical education with a jar with a bunch of red/blue or black/white beads in it. And then proceed to talk about how statistics can apply to the number of beads you might randomly select. But who carries around jars full of beads? … and who makes important business decisions based on jars of beads? Why do this? A little more thinking can quickly identify real-world scenarios that mean something to students. And THAT is when we start to remember concepts.
- Don’t confuse ‘statistics experts’ with ‘statistics gatekeepers.’ Statistics gatekeepers are those annoying people in many organizations who enjoy having the power to stop anything as opposed to help people. That is, they sit in quality, reliability or design reviews and explain how everything has been done wrong, everything needs to be more complicated, and how everyone has (once again) failed to meet their lofty expectations regarding statistics. They know enough to be intimidating to those around them. But they don’t know enough to be helpful. So they add no value, keep having their egos challenged, and are otherwise avoided. So no one is happy.
- And it starts with teaching. Chris talked about a Statistical Process Control (SPC) course he is developing which is based on breadmaking. The statistic he was using as the central example was ‘chewability.’ This sounds like a ‘made up’ abstract metric that can’t really be applied in an objective way. But it can! In fact – there are really expensive test rigs that are able to measure the hardness and elasticity of bread that is analogous to what we would describe as ‘chewy.’ The point is … everyone knows or can relate to sliced bread. And the fact that ‘chewability’ is a novel metric (at least to students) makes it interesting. So people remember it.
- But because many statistics courses aren’t fun … they aren’t done. Many undergraduate engineers manage to get their degree without ever doing a statistics course. This is a damning indictment on learning institutions. Starting with universities that employ professors who aren’t interested in crafting a message that is more interesting than jars of beads. Which one of the many reasons Accendo Reliability’s statistics articles and courses are so popular …
Enjoy an episode of Speaking of Reliability. Where you can join friends as they discuss reliability topics. Join us as we discuss topics ranging from design for reliability techniques to field data analysis approaches.
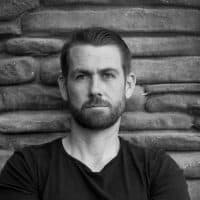
- Social:
- Link:
- Embed:
Show Notes
Thanks again for intro recording of Danzi Op. 87 No. 2 last movement. Played it last week, but not as fast.
“Statistics is Important.” Accreditation Board of Engineering and Technology requires one course in statistics; at least it used to. Engineering departments tended to offer their own statistics classes, because the real statistics courses were too tough.
Classes in AI are convenient, because AI software can replace thinking, if you have enough data. Bin Yu, UC Berkeley Statistician, and her team used AI to estimate COVID-19 daily case rates for all US counties, accounting for effects of neighboring counties. AI yields accurate, geographical baseball statistics. Bin Yu knows how to estimate survival functions. I estimated COVID-19 survival functions (aka reliability) for logistics and to infer the effects of omicron variant in South Africa and DR Congo.
Thanks Larry for your comments. I do feel like I need to share my thoughts on AI though. I think you are referring to AI in ‘machine learning’ sort of way – so I will address AI from that perspective. Firstly, (and as you point out), AI often needs a lot of data. I have not heard of too many applications where reliability engineers ever complain about having ‘too much’ data. And secondly … AI can replace thinking (as you again point out) … but that is not always a good thing. For example, we know that humidity accelerates corrosion. Temperature accelerates chemical reactions. Number of cycles accelerate fatigue failure. But … it would take AI lots and lots and lots and lots of data to be able to identify the relationships between these factors and failure modes that you can read about in many books. I have seen too many applications of AI/machine learning being applied because it seems ‘cool’ … and usually by organizations who don’t value or understand how to leverage critical thinking. For situations where we are at a loss to understand what is happening in a process … go with AI/machine learning! One could argue that DoE is like ‘machine learning lite’ … and we only do that when we are struggling to understand what is ‘really’ going on.