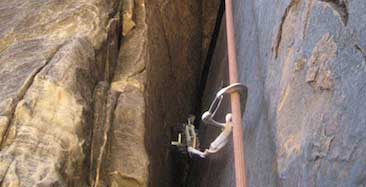
Value in being clear about reliability
One of my regular questions with clients and students is, “How do you talk about reliability, which metrics, measures, or statements do you regularly use?”
Some have learned to avoid mentioning MTBF around me. Which is fine, and if they are really using MTBF to discuss reliability then they probably know my position.
Some use statements like:
- As good as or better than the last product.
- We fix it when it fails.
- 5 years (or other duration only)
- We use whatever the customer uses.
- MTBF or a version of that.)
- Warranty, Return rate, or failure rate.
- We test to a set of industry standards.
Only occasionally does someone actually use the probability of success over a specified time period.
I maintain that we should use both probability and duration when talking about the reliability of a specific product or asset. The function and environment should be included if there is any need to avoid any confusion.
The issue with duration or probability used alone
If someone says 5 year life, they probably mean a very high chance that all the units will survive 5 years. If assuming 5 years is like an MTBF (I’ve seen this type of mis-understanding many times) then they are saying about a 36% probability of surviving 5 years is the goal.
Often that is not what they really want or meant.
If someone say 50,000 hour MTBF alone, what does that mean. Well, if that is the only bit of information, then we would use an exponential distribution and assume a constant hazard function. Meaning, each hour over the entire lifetime of the product it has a fixed 1 in 50k chance of failure.
This implies the first hour of operation and the 1 millionth hour each have the same chance to fail. It is independent of how long the unit operates.
Using just MTBF without a duration leaves us guessing as to over which time period the failure rate ( 1/ MTBF ) is valid. If the product design is for 2 years and we want to use it in an application for 10 years, this may be a very poor assumption.
Being clear leads to better decisions
I’m making a leap here based on clearer information leads to better decisions.
Using a reliability goal of make it as good as of better than the last product, when we don’t know the reliability of the last product, is not a great example of being clear.
Instead saying “we want product x used in US homes and offices to survive 5 years with 95% probability” is about a clear as you can be (may need to fill in what the product is and is supposed to do and more details around the environment).
Furthermore, we can use the same basic four part structure (function, environment, probability and duration) to state reliability goals for subsystems, modules, and components. This provide different engineering teams and vendors clear reliability goals.
When conveying the results of reliability testing – which is clearer?
– It passed the test, or
– 98% of units are expected to survive 5 years
Sure we can add lower confidence bounds on the probability when using sample data, and specify the expected environment and functions, yet, really it’s clearer.
When analyzing field data it is easy and quick to say based on returns to date, we have a 5% field failure rate. Instead maybe saying, when a product is one month old (since purchase, installation or first turn on at a customer site) 98% survive. And, at 1 year of age, 95% of units still have not failed.
A graph of field reliability is even clearer.
Providing complete reliability statements permits further analysis and comparisons. It enabled decision makers to fully understand the data and make decisions based on clear information.
Is not that a goal of any reliability task – to permit good decisions.
So, how do you talk about reliability?
A 50,000 hour MTBF (which is really a ratio of 50,000 hours per failure) implies the Reliability function, R(t), is an exponential that starts at 1.000 at 0 time and drops to approximately 0.368 at 50,000 hours. There is not a constant “chance of failure” with the exponential distribution. The “chance of failure,” the Failure Rate function, f(t), is also an exponential that starts at 20.00 parts per million (PPM) per hour at 0 time and drops to 7.36 PPM per hour at 50,000 hours. It is called the Failure Rate function because it is the ratio of failures per unit time. The “chance of failure” in the first hour is exactly 19.9998 PPM. The “chance of failure” in the thousandth hour is approximately 19.6 PPM. The “chance of failure” in the ten-thousandth hour is approximately 16.4 PPM.
The conditional probability of failure given that it hasn’t failed yet, the Hazard Rate function, h(t), is constant at 20.00 PPM per hour for as long as the exponential function is an accurate representation of the Reliability function, R(t). Possibly some wear-out failure modes begin to intrude at 10 years or 87,660 hours (figuring in leap years). Until that time, MTBF is a useful measure of reliability.