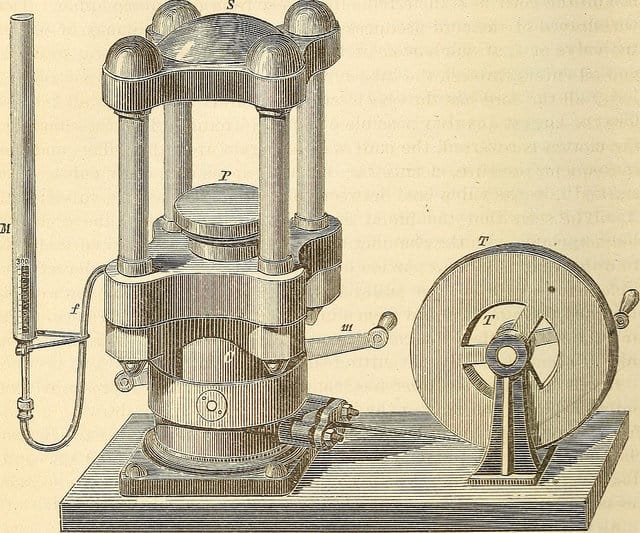
The Constant Failure Rate Myth
Have you said or have you heard someone say,
- “Let’s assume it’s in the flat part of the curve”
- “Assuming constant failure rate…”
- “We can use the exponential distribution because we are in the useful life period.”
Or something similar? Did you cringe? Well, you should have.
There are few if any failure mechanisms that actually occur with a constant hazard rate (we often even use the technically incorrect term failure rate, when talking about the instantaneous failure rate or hazard rate). The probability of failure over a short period of time now and some time in the future, say next year, is most likely going to different.
So, why do we cling to the assumed constant failure rate?
Anto Peer, Diganta Das, and Michael Pecht wrote in Appendix D, “Critique of MIL-HDBK-217” within the National Academy of Sciences book Reliability Growth: Enhancing Defense System Reliability about the nature of failure (hazard) rates. The original handbook gather data and calculated point estimates for the failure rates. Later editions of the handbook included the assumption of the generic constant failure rate model for each component. The adoption of the exponential model, which implied calculations, started in the 1950’s.
In part due to the contractual obligation to use the 217 handbook and widespread adoption of the prediction technique, the constant failure rate assumption became part of the ‘how reliability was done’. James McLinn in a paper in 1990 commented that the users of the system worked to propagate the method rather than improve the accuracy of the method. (McLinn 1990)
How do we know the failure rate changes?
Beginning in the 1950’s researchers and analysts notice component did exhibit changing failure rates. They also notices the range of failure mechanism that occurred and began modeling failure mechanisms. The work to predict failure rates based on the physical or chemical changes within a component due to applied use stress became known as physics of failure.
Numerous studies and data analysis have shown either a decreasing or increasing failure rate with time. One example is the work by Li, et.al (2008) and Patil, et.al. (2009) showing the increasing failure rate behavior for transistors.
You own data most likely shows the non-constant failure rate behavior. All you need to do is check the fit of the data to an exponential distribution to see the discrepancy.
Today we have the embedded assumption of a constant failure rate and the reality of non-constant failure rates. We also face the need to accurately describe the probability of failure based on field data, experimental data, or simulation. Simply avoiding the assumption of a constant failure rate frees us to use the information contained within time to failure data and models.
McLinn, James. 1990. Constant failure rate – A paradigm in transition? Quality and Reliability Engineering International 6:237-241.
Li, Xiaojun, Jin Qin, and Joseph B Bernstein. 2008. Compact modeling of MOSFET wearout mechanisms for circuit-reliability simulation. Device and Materials Reliability, IEEE Transactions on 8 (1): 98-121.
Patil, Nishad, Jose Celaya, Diganta Das, Kai Goebel, and Michael Pecht. 2009. Precursor parameter identification for insulated gate bipolar transistor (IGBT) prognostics. Reliability, IEEE Transactions on 58 (2): 271-276.
First of all, congratulations for the article. I have just one question:
You know the work done by Nolan and Heap regarding the failure patterns that they found when investigating why aircraft maintenance strategies could not reduce the accident rate.
They showed that more than 89% of the components presented a constant failure rate pattern and just a very small portion of infant mortality and wear out patterns.
What is your opinion about it, because this is always remembered when I say that constant failure rate is rarer than win a national lottery
Hi William,
Yes very familiar with the Nolan and Heap report. Keep in mind that they were tracking data based on heavily replaces and refurbished equipment. Given the conservative nature of keeping aircraft flying they rarely waited for wear out.
It is data from an interesting dataset, yet I really would like to see the raw data and how they did the analysis.
I’ve yet to see anything that is truly following the exponential distribution.
Sometimes a system or component is close, yet often only over a very select time period, often short.
Cheers,
Fred
Thank you for the prompt answer. I’m one of the No MTBF warriors in Brazil.
Thanks for the support and do let me know if you run across any other champions, good stories, or gnarly obstacles.
Thanks for the comment, too.
Cheers,
Fred
I read the same document and working in the aviation industry, MTBF is one or should say the preferred metric. I’ve seen overhaul scheduled based on MTBF. When I questioned their methodology I was told it was perfectly fine to use this metric. I’m not not a big fan of the MTBF but changing the culture is quite a challenge.
If we don’t try to change the culture, it most likely won’t change. Keep on asking and pushing forward better methods. We’ll get there eventually. We just have to keep working to eradicate the mis use of MTBF.
Cheers,
Fred
I wonder if it is a situation explained by Drenick’s theorem. Lump enough failure modes together, and the group appears to be random. This is easy to imagine when the levels of stress are low enough to cause very slow wear-out, as would be expected in a well designed system.
Hi Merrill,
If one is really not interested in the mechanisms and there are plenty of them, at times the system failures may appear random…not a useful approach to monitor and improve a system (or even maintain).
If the system is well designed and there exists a slow wear out mechanism, then conduct a specific ALT for that mechanism. Go as slow or fast as the mechanism dictates.
Cheers,
Fred
Fred,
You make a very good comment. Every failure has a physical cause. If I never exceed the stress (voltage, current, thermal, etc.) corresponding to the strength of the weakest component then it won’t fail. Of course, the strength of the weakest component degrades over time. This means that if the distribution of the stresses on a component or assembly doesn’t vary with time, then the probability of failure will increase with time. Nolan and Heap provide a good model of this (section 2.5).
There are two points relevant to the Nolan and Heap report. First, brakes and tires wear out on individual planes, but for fleet maintenance the rate at which spares are purchased will may well appear to be constant due to choice of averaging times and the fact that units have different ages. As you have rightly pointed out before, choosing the wrong model or misapplying the right model generally leads to wrong conclusions.
Also, Heap and Nolan do use life data analysis and condition based replacements in addition to scheduled replacements (although I’d imagine that maintenance policies have also changed since 1978). I’d think that they could well be measuring the onset of wear out, and that quality control prevents most of the infant mortality problem. That amounts to heavily censored data, I should think, and it’s possible (as you note) to fit a constant rate model to a portion of the data.
There’s a great opportunity to test all of this with Boeing’s latest real-time performance based maintenance program for the Dreamliner. As you point out, understanding the analysis is critical. One shift that I think is important is the idea that failure isn’t just that a component turns into a pile of dust. If failures are defined in terms of not meeting performance requirements rather than “off,” then a component can be “working.” This notion is already part of most people’s experience: a tire is replaced when the remaining tread is less than some minimum, and not when it can no longer hold air. I don’t know if there are any reports or papers out yet. Do you know of any?
Nice Article Fred,
I’ve in mind and instruct that when constant failure rate is found some mixture os failure modes are being analysed together. Thus some effort previous the analysis should take place where a RCA methology can be applied in order to help the analist understands how all the failure modes are being caused. Having this handled the organization can clean up all “external” causes that’s leading to the especific failure mode and perform the analysis with accurately.
Thanks Francilei, good point and approach. All too often folks just want to assume the constant failure rate and look no further. Keep up the good work. Cheers,
Fred
I’ve been a Reliability Professional within the DoD for some years and have been strictly confined to MTBF as the metric of choice. Over the years I’m come to believe that statistical data (in general not just in reliability) is of use only when the mechanisms are not understood or too complicated to model based on physical attributes. I think of the statistical measures of reliability as a sort of filler between the physics, or a glue between the understood and not-understood (or not known) physics in the model. Nonetheless, in the DoD world I have to translate those physics back into a MTBF because it is the metric that I am required to use to report my findings back to the DoD.
Hi David,
So sad that you are ‘required’ to use MTBF.
I would say that statistics helps us to model and deal with the very real and physical variation that occurs with every item and situation we encounter. It’s not a crutch to bridge that we know with what we do not know. Statistics is the language of variation. It allows us to describe and model elements that are not practical to model in detail. We do not need to model the grain structure in every PN junction to perfectly model diffusion which leads to failure – we know it exists and can use statistics to describe the variability of the time to failure for that failure mechanism. No physics of failure model perfectly models any physical process and has the need to use the language of variability along the way.
MTBF – is a choice and often obscures and hides the real information in a set of data. I would recommend you and with the help of the NoMTBF community push back on having to use MTBF. At the minimum send informative results and MTBF and highlight the difference – the very real differences that lead to faulty decisions.
May be making an assumption that those in the DOD want to make good decisions.
alas,
Fred
Fred, thanks for the feedback. We may be saying the same thing in different ways, but let me attempt to further clarify my position on the use of statistics using your PN example. Statistics provides an ability to understand circumstances in aggregate; this would include the life of a PN junction for which the physics is pretty well understood. In this case we – with eyes wide open – choose to simplify the evaluation of the PN junction to a statistical value. This approach provides utility in several ways for instance to develop meaningful predictions of entire systems of components. However, the use of a statistic can also be masking a lack of understanding of the physics and therefore provides a crutch. For example, one may derive an MTBF through testing that remains accurate throughout the lifecycle without ever understanding the physical drivers of that MTBF. Or, we may choose to use the statistical value because there is something we don’t know yet, but still need to move forward with the analysis. I do believe in the use of statistics, but I think they can be easily misused.
I will look into the NoMTBF discussions and will of course take every opportunity to improve on DoD processes.
Hi David,
As you suspected we agree more than not. Yes stats is just a tool and given the current state of awareness and understanding of stats by many, it is often mis-used.
Good luck with changing the culture at DOD around MTBF – you are not alone as I’ve run across many via this blog that are also frustrated and working to improve the situation.
Cheers,
Fred
Your article popped up when I searched for articles on constant failure rates. So I cited your article in mine, “Reliability Management of Failure Rates, How to get a Constant Failure Rate in Calendar TIme,” https://sites.google.com/site/fieldreliability/would-you-like-constant-failure-rate, so that people could have a choice. There is some legitimate motivation for a constant, calendar-time failure rate: “demand leveling.”
Thanks Larry, might be wining this one with the help of Google….
Cheers,
Fred