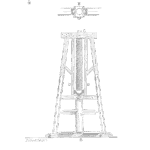
The trite answer is just as many samples as you need and not one more.
A better answer is enough samples to make the right decision. The realistic answer is you will not enough samples.
“How many samples?” is an oft-asked question when planning for quality or reliability testing.
In some cases, a sample may take months to create and are expensive.
In other cases, you will have to deal with mock up or partially function samples.
In all cases, the samples you need for reliability testing will have to provide value.
The investment in samples expects the return of knowledge.
You need to convert those sparse group of units into valuable information revealing what will fail or when failures will occur.
So, how do you estimate how many samples you need for a specific experiment?
To determine the necessary sample size you need three pieces of information.
The risk, the variance, and the precision. Let’s discuss each of these in a little more detail.
The risk
There are two kinds of risk here: the risk of the unknown and the statistical risk.
Risk of the unknown
The first type concerns not doing the experiment. Making a decision without the data.
The chance the experiment will reveal vital information or confirm a hypothesis.
This risk of the unknown also includes unknown errors in the samples or test process.
The testing itself may produce faulty (often hidden) results.
If you do not carefully design the experiment the results have a chance of being bogus.
Garbage in, garbage out. And this risk has little to do with the sample size.
Statistical risk
The statistical risk involves the sample size directly.
There is a statistical chance that the randomly selected set of a unit for the sample are generally better than the population or vice versa.
The basis for statistical testing and using a random sample is the sample will represent the population. Alas, there is a chance it will not.
We use the term statistical confidence, or type I and type II error, or power, to describe the ability to the sample to properly represent the population.
For example, a 95% confidence means that associated sample as a 5% chance of misrepresenting the population resulting in the analysis producing a false conclusion.
The statistical risk that is acceptable is a policy decision.
The variance
Variation happens.
Variance is the statistical measure of variability in a population of data.
It is what it is and the only way to reduce variability is the change the product design or production process.
A large variance will require a higher sample size to detect a shift in results of an experiment.
We often do not know the population variance and have to estimate the variance elements using the sample data.
The precision
This is related to what we are trying to detect.
If the new design has more strength than the old design, then how much stronger does the new design have to be to be worth noticing.
If we want to detect a one gram increase in average load bearing capability, we would require many more samples than if we only wanted to detect a kilogram difference.
The larger the difference that we desire to detect the fewer samples we will require.
This sample size changes as it is more difficult to conclusively detect a small change.
We may measure one sample from the old and new designs and see a difference, maybe even a very small difference.
Yet does this represent the actual shift and amount of shift in the overall population?
Most likely not.
On the other hand, if we measure a new sample and it is 1,000 times stronger, statistically speaking we can be pretty sure the new design is stronger.
This is rarely the case and we need to detect smaller changes, thus more samples.
Summary
Most sample size formulas contain these three elements.
Two parts, risk and precision, are business or technical decisions. The variance is part of the underlying data and not an option to increase or decrease easily.
The sample size formula is useful for the discussion around risk and decision points prior to conducting the experiment.
It is one way to design and conduct measurements that provide value.
If our results are to be useful than considering all three elements that make up a sample size formula become important.
Related:
Sample Size Success Testing (article)
Sample Size (article)
Three Considerations for Sample Size (article)
Leave a Reply